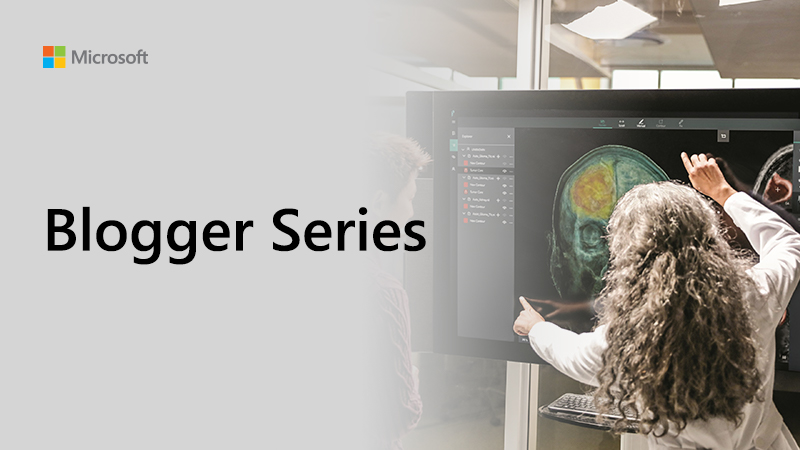
Changing lives with AI in healthcare
Antonio is a Principal Researcher at Microsoft Research in Cambridge, and he leads Project InnerEye, a research project that uses machine learning technology for assistive cancer treatment.
In 2000 Antonio obtained his doctorate degree (PhD) in computer vision from the University of Oxford. He has written and co-authored numerous scientific papers and books on machine learning for the analysis of radiological images, decision forests, deep learning and convolutional neural networks, object recognition, image and video analysis and editing, videoconferencing, 3D reconstruction and virtual/augmented reality, forensic science and history of art. Antonio’s research has been awarded a number of prizes including the prestigious David Marr prize at ICCV 2015 for his paper “Deep Neural Decision Forests”.
Cancer affects us all, whether that’s directly or indirectly, and we all know treatments such as chemotherapy or radiotherapy can have terrible side effects. Here at Microsoft Research Cambridge we’re using AI and machine learning to assist medical practitioners and potentially improve the lives of cancer patients.
I lead Project InnerEye, which looks at the application of machine learning for the analysis of medical images, turning multi-dimensional radiological images into measuring devices. This project builds upon many years of research in computer vision and machine learning and employs algorithms such as deep decision forests and convolutional neural networks.
In Project InnerEye, we are developing a technology that is designed to work alongside the experts to actively predict and find tumours and healthy tissues in a 3D radiological image.
The hope is for our technology to help the world of cancer treatment to move towards adaptive radiotherapy.
We aim to improve the efficiency and effectiveness of cancer treatments while reducing costs for the healthcare providers, and that’s why I’m so passionate about the work we’re doing.
How does Project InnerEye do this? Traditionally the process of identifying tumours in these images is very time consuming and expensive. A health practitioner may spend hours going through individual cross sections, manually delineating tumours and healthy organs. These images eventually come together to become a 3D image and then the practitioner can decide on a treatment plan. InnerEye will identify tumours and healthy tissues in 3D scans in a matter of minutes, allowing for faster planning, more targeted therapy, and precise surgery planning and navigation.
One of the main ways of treating cancer is through radiotherapy, where ionising radiation is targeted to a specified region in the patient’s body. The radiation destroys the DNA of the tumour cells and it is vital to know exactly the boundaries of nearby healthy anatomy, so we can preserve healthy cells.
Since the planning process is so time consuming, what tends happens is that planning is done only once, at the beginning of radiotherapy, even though the therapy itself is delivered over different sessions for up to 40 days.
That means that over these sessions there is no account for any changes that could happen such as the tumour shrinking, weight loss or other anatomical changes.
The hope is that InnerEye could help health practitioners do the planning before each treatment fraction, thus adapting to the changing shape of the tumour and the patient’s anatomy.
Perhaps this will lead to needing fewer fractions (e.g. 5 instead of 40), while reducing costs and hopefully improving outcomes.
The aim of our research is to use machine learning to assist the experts. In fact, the expert practitioner can always refine and adjust the proposed segmentations, keeping control of the final quality at all times.
InnerEye is not a diagnostic tool. While health practitioners are effective at diagnosing conditions from images, what they need is better quantification tools. InnerEye produces segmentation of regions of interest, which in turn makes quantification much easier.
We have and continue to gather anonymised images with consent from clinical institutions globally. Being able to train our algorithm with all those images from different geographies and different body types ensures our technology will work globally rather than just in the UK.
While we spend most of our work concentrating on cancer, with the technology helping to identify solid tumours in the brain, liver, pancreas, lungs, kidneys, and many other areas, it has many other applications.
In theory InnerEye could help delineate other anomalies such as aneurysms or multiple sclerosis plaques for instance, showing there is a wide scope for this technology in the future.
Take a listen to our podcast to find out more about how we’re changing lives in healthcare using AI and machine learning.
Listen to the podcast: Changing lives in healthcare using AI
The InnerEye cloud API is designed to integrate within 3rd party medical software and is already being used by a number of clinical institutions globally where it is being actively used and delivering good results. If you’re interested in integrating InnerEye into your software, email InnerEyeInfo@microsoft.com.