Keynote
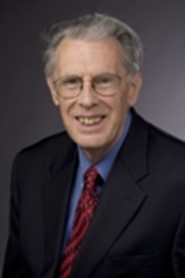
John Hopcroft
Cornell University
Talk title: Theory in an industrial lab
-
This talk will discuss the role of a theory group at MSRA and then focus on some research that might be important to Microsoft.
-
John E. Hopcroft is the IBM Professor of Engineering and Applied Mathematics in Computer Science at Cornell University. He received his BS (1961) from Seattle University, his M.S. (1962) and Ph.D. (1964) in EE from Stanford University. He is a member of the National Academy of Sciences, of the National Academy of Engineering, and a foreign member of the Chinese Academy of Sciences. In 1992, President George Bush appointed him to the National Science Board, which oversees the National Science Foundation, and served through May 1998. He has received numerous awards and honorary degrees including the Turing Award in 1986. In 2016, Premier Li Keqiang presented him with the Friendship Award for his work in China.

Terry Lyons
University of Oxford
Talk title: From mathematics to data science and back
-
Contextual framing, empirical validation, and mathematical innovation are three important elements of research; they have different timescales and may not fit comfortably together. Even so, major developments can require all three.
The pressure to deliver accurate inference from streamed data at low energy leads one to connect practical challenges with mathematics that (initially) seems very far from the world of recurrent neural nets and streamed data. We will explain some small window of connections – and demonstrate that the benefits are bidirectional – with new mathematics as well as new data science.
-
Professor Terry Lyons is the Wallis Professor Emeritus and Professor of Mathematics. He is currently Principal Investigator of the DataSıg program (primarily funded by EPSRC), and of the complementary research programme CIMDA-Oxford. He was a founding member (2007) of, and then Director (2011-2015) of, the Oxford Man Institute of Quantitative Finance; he was the Director of the Wales Institute of Mathematical and Computational Sciences (WIMCS; 2008-2011). He came to Oxford in 2000 having previously been Professor of Mathematics at Imperial College London (1993-2000), and before that he held the Colin Maclaurin Chair at Edinburgh (1985-93).
Professor Lyons’s long-term research interests are all focused on Rough Paths, Stochastic Analysis, and applications – particularly to Finance and more generally to the summarising of large complex data. More specifically, his interests are in developing mathematical tools that can be used to effectively model and describe high dimensional systems that exhibit randomness as well as the complex multimodal data streams that arise in human activity. Professor Lyons is involved in a wide range of problems from pure mathematical ones to questions of efficient numerical calculation. DataSıg is a project that bridges from the fundamental mathematics to application contexts where novel techniques for analysing streamed data have potential to contribute value; these contexts include mental health, action recognition, astronomy, cyber-security, … The CIMDA-Oxford research partnership aims to address a broader range of mathematical and engineering challenges arising from multi-dimensional big data.
Panel
Panelists
Xiaotie Deng
Peking University
-
Xiaotie Deng got his BSc from Tsinghua University, MSc from Chinese Academy of Sciences, and PhD from Stanford University in 1989.
He is currently a chair professor at Peking University. He taught in the past at Shanghai Jiaotong University, University of Liverpool, City University of Hong Kong, and York University. Before that, he was an NSERC international fellow at Simon Fraser University. Deng’s current research focuses on algorithmic game theory, with applications to Internet and Blockchain Economics and Finance. His other works cover online algorithms, parallel algorithms and combinatorial optimization.
He is an ACM fellow for his contribution to the interface of algorithms and game theory, an IEEE Fellow for his contributions to computing in partial information and interactive environments, and a CSIAM Fellow for contributions in game theory and blockchain. He is a foreign member of Academia Europaea.
Pinyan Lu
Shanghai University of Finance and Economics
-
Pinyan Lu is a professor and the founding director of Institute for Theoretical Computer Science at Shanghai University of Finance and Economics (ITCS@SUFE). Before joining SUFE, he was a researcher at Microsoft Research. He studied in Tsinghua University (BS (2005) and PhD (2009) both in Computer Science). He is interested in theoretical computer science, including complexity theory, algorithms design and algorithmic game theory. Currently, his research is mainly focused on complexity and approximability of counting problems, and algorithmic mechanism design.
Pinyan is the recipient of a number of awards including ICCM Silver Medal of Mathematics, Distinguished Membership of ACM and CCF, CCF Young Scientist award, Best Paper Award from ICALP 2007, FAW 2010, ISAAC 2010 and so on. He is the PC chairs for FAW-AAIM 2012, WINE 2017, FAW 2018, ISAAC 2019 and so on, and PC members for STOC, FOCS, SODA and a dozen of international conferences. He is an Editorial Board Member of the “Information and Computation” and “Theoretical Computer Science”.
Liwei Wang
Peking University
-
Liwei Wang is a professor and Vice Dean of Department of Machine Intelligence, School of Electronics Engineering and Computer Sciences, Peking University. His main research interest is machine learning theory. He has published more than 150 papers on top conferences and journals. He is an Associate Editor of TPAMI. He served as Area Chair of NeurIPS, ICML and ICLR for many years. He was named among “AI’s 10 to Watch”.
Wei Chen
Microsoft Research Asia
-
Wei Chen is a Principal Researcher at Microsoft Research Asia, and an Adjunct Researcher at Chinese Academy of Sciences and Shanghai Jiao Tong University. He is a standing committee member of the Technical Committee on Theoretical Computer Science, Chinese Computer Federation, and a member of the CCF Technical Committee on Big Data. He is a Fellow of Institute of Electrical and Electronic Engineers (IEEE). He is selected as one of the world’s top 2% scientists by a Stanford University ranking. Wei Chen’s main research interests include online learning and optimization, social and information networks, network game theory and economics, distributed computing, and fault tolerance. He has done influential research on the algorithmic study of social influence propagation and maximization, combinatorial online learning, and fault-tolerant distributed computing, with 13000+ collective citations on these topics. He has one coauthored monograph in English in 2013 and one sole authored monograph in Chinese in 2020, both on information and influence propagation in social networks. His research papers have won a number of awards, including the 10-Year Highest Impact Paper Award at ICDM’2021, Best Student Paper at ECML-PKDD 2010, and the William C. Carter Award at DSN’2000. He has served as editors, academic conference chairs and program committee members for many academic conferences and journals. Wei Chen has Bachelor and Master degrees from Tsinghua University and a Ph.D. degree in computer science from Cornell University. For more information, you are welcome to visit his home page at http://approjects.co.za/?big=en-us/research/people/weic/.
Wei Chen
Microsoft Research Asia
-
Wei Chen (陈薇) is a principal research manager in Microsoft Research Asia. She is leading the Computing and Learning Theory Group (opens in new tab). Her current research interests include deep learning theory, trustworthy machine learning, and distributed machine learning. Before joined Microsoft, she obtained her Ph.D. in Math from Chinese Academy of Sciences, advised by Prof. Zhi-Ming Ma (opens in new tab). Now, she is an adjunct professor in the University of Science and Technology of China, Beijing Jiaotong University, and Nankai University.
Moderator
Tie-Yan Liu
Microsoft Research Asia
-
Tie-Yan Liu is an assistant managing director of Microsoft Research Asia, leading the machine learning research area. He is a fellow of the IEEE and a distinguished member of the ACM. He is an adjunct professor at Carnegie Mellon University (CMU), Tsinghua University (THU), Nankai University, and University of Science and Technology of China (USTC), and an honorary professor at Nottingham University.
Talks
Jian Li
Tsinghua University
Talk title: Theoretical Aspects of Gradient Methods in Deep Learning
-
Deep learning has enjoyed huge empirical success in recent years. Although training a deep neural network is a highly non-convex optimization problem, simple (stochastic) gradient methods are able to produce good solutions that minimize the training error, and more surprisingly, can generalize well to out-of sample data, even when the number of parameters is significantly larger than the amount of training data. It is known that changing the optimization algorithm, even without changing the model, changes the implicit bias, and also the generalization properties. What is the bias introduced by the optimization algorithms for neural networks? What ensures generalization in neural networks? In this talk, we discuss recent theoretical work on the above questions.
-
Jian Li is currently an (tenured) associate professor at Institute for Interdisciplinary Information Sciences (IIIS), Tsinghua University, headed by Prof. Andrew Yao. He got his BSc degree from Sun Yat-sen (Zhongshan) University, China, MSc degree in computer science from Fudan University, China and PhD degree in the University of Maryland, USA. His major research interests lie in theoretical computer science, Machine learning, databases. Recently, he is interested in learning theory, and applying machine learning to financial applications. He co-authored several research papers that have been published in major computer science conferences and journals. He received the best paper awards at VLDB 2009 and ESA 2010, best newcomer award at ICDT 2017.
Yuan Zhou
BIMSA
Talk title: Batch Online Learning and Decision-Making
-
Motivated by practical needs such as large-scale learning, we study the impact of the adaptivity constraints to online learning and decision-making problems. Unlike traditional online learning problems which allow full adaptivity at the per-time-step scale, our work investigates the models where the learning strategy cannot frequently change and therefore enables the possibility of parallelization.
In this talk, I will focus on batch learning, a particular learning-with-limited-adaptivity model, and show that only O(log log T) batches are needed to achieve the optimal regret for the popular linear contextual bandit problem. Along the way in the proof, I will also introduce the distributional optimal design, a natural extension of the optimal experiment design in statistical learning, and introduce our statistically and computationally efficient learning algorithm for the distributional optimal design, which may be of independent interest.
I will also briefly mention our (very) recent process on the tight batch-regret trade-off for linear bandits, and our on-going work on batch learning for Markov decision processes.
-
Yuan Zhou is a Research Associate Professor at the Yanqi Lake Beijing Institute of Mathematical Science and Applications (BIMSA). His research interests include stochastic and combinatorial optimizations and their applications to machine learning and operations research & management. He is also interested in and publishes on analysis of mathematical programming, approximation algorithms, and hardness of approximation.
Hu Fu
Shanghai University of Finance and Economics
Talk title: Sequential Search Problems Beyond The Pandora Box Setting
-
Weitzman in 1979 studied the Pandora Box as a model of consumer’s sequential search procedure, and gave an elegant optimal algorithm. The algorithm was later understood to be a special case of the celebrated Gittins index algorithm for Bayesian bandits.
Algorithmic interests in Pandora Box problem are reviving in recent years, due to the problem’s connection to mechanism design and other online decision problems. I will review some extensions of the model, to combinatorial settings and to relaxations of the original model, and mention recent progress on these fronts.
-
Hu Fu is an associate professor at the Institute for Theoretical Computer Science, Shanghai University of Finance and Economics. He earned his PhD in Computer Science from Cornell University, and was a postdoc at Microsoft Research New England lab and at Caltech. Before joining SHUFE, he was an assistant professor at University of British Columbia. His research interests include mechanism design, algorithmic game theory and online algorithms.
Shaofeng Jiang
Peking University
Talk title: Coresets for Clustering with Missing Values
-
We provide the first coreset for clustering points in $mathbb{R}^d$ that have multiple missing values (coordinates). Previous coreset constructions only allow one missing coordinate. The challenge in this setting is that objective functions, like $k$-Means, are evaluated only on the set of available (non-missing) coordinates, which varies across points. Recall that an $epsilon$-coreset of a large dataset is a small proxy, usually a reweighted subset of points, that $(1+epsilon)$-approximates the clustering objective for every possible center set.
Our coresets for $k$-Means and $k$-Median clustering have size $(jk)^{O(min(j,k))} (epsilon^{-1} d log n)^2$, where $n$ is the number of data points, $d$ is the dimension and $j$ is the maximum number of missing coordinates for each data point. We further design an algorithm to construct these coresets in near-linear time, and consequently improve a recent quadratic-time PTAS for $k$-Means with missing values [Eiben et al., SODA 2021] to near-linear time.
We validate our coreset construction, which is based on importance sampling and is easy to implement, on various real data sets. Our coreset exhibits a flexible tradeoff between coreset size and accuracy, and generally outperforms the uniform-sampling baseline. Furthermore, it significantly speeds up a Lloyd’s-style heuristic for $k$-Means with missing values.Joint work with Vladimir Braverman, Robert Krauthgamer and Xuan Wu.
-
Shaofeng Jiang is an assistant professor and a Boya Young Fellow at Center on Frontiers of Computing, Peking University. He obtained his Ph.D. from the University of Hong Kong, and before he joined PKU, he worked as a postdoctoral researcher at the Weizmann Institute of Science and then an assistant professor at Aalto University. His research interest is generally theoretical computer science, with a focus on algorithms for massive datasets, online algorithms and approximation algorithms.
Longbo Huang
Tsinghua University
Talk title: What Makes Multi-modal Learning Better than Single (Provably)
-
Recently, joining the success of deep learning, there is an influential line of work on deep multi-modal learning, which has remarkable empirical results on various applications. However, theoretical justifications in this field are notably lacking.
Can multi-modal learning provably perform better than uni-modal?
In this work, we answer this question under a most popular multi-modal fusion framework, which firstly encodes features from different modalities into a common latent space and seamlessly maps the latent representations into the task space. We prove that learning with multiple modalities achieves a smaller population risk than only using its subset of modalities. The main intuition is that the former has a more accurate estimate of the latent space representation. To the best of our knowledge, this is the first theoretical treatment to capture important qualitative phenomena observed in real multi-modal applications from the generalization perspective. Combining with experiment results, we show that multi-modal learning does possess an appealing formal guarantee.
-
Dr. Longbo Huang is an associate professor (with tenure) at the Institute for Interdisciplinary Information Sciences (IIIS) at Tsinghua University, Beijing, China. Dr. Huang has held visiting positions at the LIDS lab at MIT, the Chinese University of Hong Kong, Bell-labs France, Microsoft Research Asia (MSRA), and the Simons Institute for the Theory of Computing at UC Berkeley. Dr. Huang serves/served on the TPCs and organizing committees for IEEE/ACM/AI conferences, including the General Chair for ACM Sigmetrics 2021, the TPC co-chair for ITC 2022, WiOpt 2020 and NetEcon 2020. Dr. Huang serves on the editorial board for ACM Transactions on Modeling and Performance Evaluation of Computing Systems (ToMPECS) and IEEE/ACM Transactions on Networking (ToN). Dr. Huang received the Outstanding Teaching Award from Tsinghua university in 2014, the Google Research Award and the Microsoft Research Asia Collaborative Research Award in 2014, and was selected into the MSRA StarTrack Program in 2015. Dr. Huang won the ACM SIGMETRICS Rising Star Research Award in 2018.
Wang Miao
Peking University
Talk title: Causal inference, observational studies, and the 2021 Nobel Prize in Economics
-
This year’s Nobel prize in economics is awarded to Card, Angrist and Imbens for their contributions to causal inference in observational economic studies. The motivations of the Nobel economics prize in 1989 and 2000 were also closely related to causal inference. I will briefly go through the scientific backgrounds of this year’s and 1989 and 2000’s Nobel economics prize. In fact, these works are paralleled by researches in statistics, which is a predominant field for causal inference research. I will roughly review the statistical contributions that are undervalued, and discuss recent progress and possible integration with AI.
-
I am currently Assistant Professor at the Department of Probability and Statistics at Peking University. I have been Assistant Professor at Guanghua School of Management in 2018-2020. I obtained PhD (2017) and BS(2012) degrees from the School of Mathematical Sciences at Peking University, and did postdoctoral research at the Department of Biostatistics at Harvard University. My research interest spans in causal inference, missing data analysis, semiparametrics, and their application in epidemiology, biostatistics, economics and AI. My website—https://www.math.pku.edu.cn/teachers/mwfy/ (opens in new tab)
Qi Meng
Microsoft Research Asia
Talk title: The implicit bias of optimization algorithms in deep learning
-
Despite their overwhelming capacity to overfit, deep neural networks trained by specific optimization algorithms tend to generalize well to unseen data. Recently, a remarkable progress to explain generalization of deep learning algorithms shows that gradient descent converges to max margin solution on homogeneous neural networks. We extend it to optimizers with momentum and pre-conditioning.
-
Qi Meng is a senior researcher in Computational and Learning Theory Group (COLT) in Microsoft Research Asia (MSRA). Before she joined Microsoft in July 2018, she obtained her Ph.D. in probability and mathematical statistics from Peking University. Her main research interests include machine learning theory and learning dynamics.
Xiangchan Zhu
Chinese Academy of Science
Talk title: Recent results on stochastic 3D Navier-Stokes equations
-
We will recall recent results on the three dimensional incompressible Navier-Stokes equations driven by stochastic forcing. First, for every divergence free initial condition in L2, we obtain existence of innitely many global-in-time probabilistically strong and analytically weak solutions, solving one of the open problems in the field. This result in particular implies non-uniqueness in law. Second, we give non-uniqueness of the associated Markov processes in a suitably chosen class of analytically weak solutions satisfying a relaxed form of an energy inequality. Translated to the deterministic setting, we obtain non-uniqueness of the associated semi flows.
-
Xiangchan Zhu is a professor in Chinese Academy of Science. Her main research interest is stochastic partial differential equations (SPDE), including singular SPDE, stochastic Navier-Stokes equation, KPZ equation, stochastic quantization equation and stochastic Euler equation.