À propos
I am a senior principal researcher at Microsoft Research, New York City (opens in new tab). Previously, I spent two years as an assistant professor in the College of Information and Computer Sciences at the University of Massachusetts, Amherst and a year as a postdoctoral researcher at Microsoft Research, NYC. Before that, I completed my PhD in the Computer Science Department at Carnegie Mellon University, advised by Aarti Singh (opens in new tab). I received my undergraduate degree in EECS at UC Berkeley.
My research interests are in machine learning and statistics. I am most excited about interactive learning, or learning settings that involve feedback-driven data collection. My recent interests revolve around decision making problems with limited feedback, including contextual bandits and reinforcement learning.
For a list of preprints and papers, please visit my personal webpage (opens in new tab).
Microsoft Research Podcast & Blog
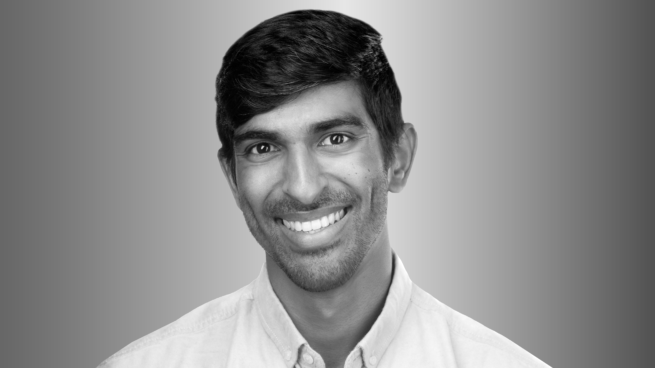
Provably efficient reinforcement learning with Dr. Akshay Krishnamurthy
Episode 117 | June 3, 2020 - MSR’s New York City lab is home to some of the best reinforcement learning research on the planet but if you ask any of the researchers, they’ll tell you they’re very interested in getting it out of the lab and into the real world. One of those researchers is Dr. Akshay Krishnamurthy and today, he explains how his work on feedback-driven data collection and provably efficient reinforcement learning algorithms is helping to move the RL needle in the real-world direction.

Provably efficient reinforcement learning with rich observations
Reinforcement learning, a machine learning paradigm for sequential decision making, has stormed into the limelight, receiving tremendous attention from both researchers and practitioners. When combined with deep learning, reinforcement learning (RL) has produced impressive empirical results, but the successes to…