À propos
I am a researcher in the AI Frontiers (opens in new tab) lab at Microsoft Research (opens in new tab). My research work lies in the intersection of human and machine intelligence aiming at improving current systems either with better debugging tools or by optimizing them for human-centered properties. I am currently excited about two main directions in this intersection:
Debugging and Failure Analysis of AI\ML Systems for accelerating the software development lifecycle of reliable and robust learning systems. I build tools that enable machine learning practitioners identify and diagnose failures in learned models. Take a look at Error Analysis (opens in new tab) and BackwardCompatibilityML (opens in new tab) as examples of such tools in the open source community.
Human-AI Collaboration for enhancing human capabilities while solving complex decision-making tasks. I study properties of ML models that make them better collaborators with people and design new optimization techniques encode such properties into models. Check out my recent talk on this topic: The Utopia of Human-AI Collaboration. (opens in new tab)
I am also involved in various research initiatives that study the societal impact of artificial intelligence as well as various quality-of-service aspects of AI including interpretability, reliability, accountability, and fairness.
If you are a PhD student looking for an internship position around these topics send me an email. We are currently pursuing research on understanding and evaluating foundation models. Example topics include mechanistic interpretability, tools for failure understanding and model comparison, data synthesis for training and evaluation, methods and benchmarks for evaluating fundamental and generative capabilities of large foundation models.
Prior to joining Microsoft Research, in 2016 I completed my PhD degree at ETH Zurich (opens in new tab) (Switzerland) in the Systems Group (opens in new tab), advised by Prof. Donald Kossmann (opens in new tab) and Prof. Andreas Krause (opens in new tab). In 2011, I completed my master studies in computer science in a double-degree MSc program at RWTH University of Aachen (opens in new tab) (Germany) and University of Trento (opens in new tab) (Italy) as an Erasmus Mundus (opens in new tab) scholar. I also have a Diploma in Informatics (opens in new tab)from University of Tirana (opens in new tab) (Albania) from where I graduated in 2007.
Featured Content

EUREKA: Evaluating and Understanding Large Foundation Models
Rigorous and reproducible evaluation of large foundation models is critical for assessing the state of the art, informing next steps in model improvement, and for guiding scientific advances in Artificial Intelligence (AI). Evaluation is also important for informing the increasing…

Responsible AI: The research collaboration behind new open-source tools offered by Microsoft
As computing and AI advancements spanning decades are enabling incredible opportunities for people and society, they’re also raising questions about responsible development and deployment. For example, the machine learning models powering AI systems may not perform the same for everyone…

Responsible AI Mitigations and Tracker: New open-source tools for guiding mitigations in Responsible AI
The goal of responsible AI is to create trustworthy AI systems that benefit people while mitigating harms, which can occur when AI systems fail to perform with fair, reliable, or safe outputs for various stakeholders. Practitioner-oriented tools in this space help with accelerating the model improvement lifecycle from identification to diagnosis and then mitigation of responsible AI concerns. This blog describes two new open-source tools in this space developed at Microsoft Research as part of the larger Responsible AI Toolbox(opens in new tab) effort in collaboration with Azure Machine Learning and Aether, the Microsoft advisory body for AI ethics and effects in engineering and research: Responsible AI Mitigations library(opens in new tab) – Python library for implementing and exploring mitigations for Responsible AI. Responsible AI Tracker(opens in new tab) – JupyterLab extension for tracking, comparing, and validating Responsible AI mitigations and experiments.

Responsible Machine Learning with Error Analysis
We are happy to share the Error Analysis tool with the open source community. The tool is based on our earlier work for failure explanation in ML systems and was developed with the help of our amazing partners in Azure Machine Learning and Microsoft Mixed Reality.
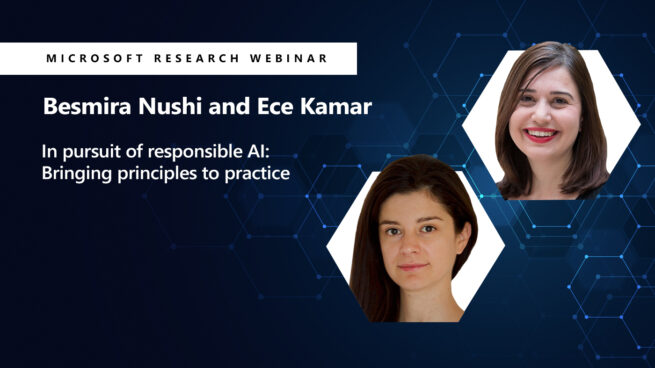
In pursuit of responsible AI: Bringing principles to practice webinar
The webinar will present examples of how these learnings are shaping our research on developing principles and tools for bringing the AI principle of reliability and safety to reality. In particular, it will showcase an ecosystem of open-source tools that are intended to accelerate the machine learning (ML) development life cycle by identifying and mitigating failures in a faster, systematic, and rigorous way.

Adaptive systems, machine learning and collaborative AI with Dr. Besmira Nushi
Episode 102 | December 11, 2019 - With all the buzz surrounding AI, it can be tempting to envision it as a stand-alone entity that optimizes for accuracy and displaces human capabilities. But Dr. Besmira Nushi, a senior researcher in the Adaptive Systems and Interaction group at Microsoft Research, envisions AI as a cooperative entity that enhances human capabilities and optimizes for team performance. On the podcast, Dr. Nushi talks about what it takes to develop collaborative AI systems and unpacks the unique challenges machine learning engineers face in their version of the software development cycle. She also reveals why understanding the “terrain of failure” can help researchers develop AI systems that perform as well in the real world as they do in the lab.

Creating better AI partners: A case for backward compatibility
Artificial intelligence technologies hold great promise as partners in the real world. They’re in the early stages of helping doctors administer care to their patients and lenders determine the risk associated with loan applications, among other examples. But what happens…