Presented by Naoto Usuyama at Microsoft Research Forum, March 2024
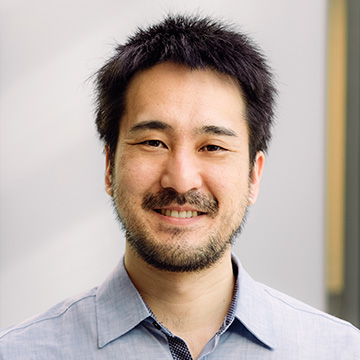
“This project (GigaPath) is not possible without many, many collaborators, and we are just scratching the surface, so I’m very excited, and I really hope we can unlock the full potential of the real-world patient data and advance AI for cancer care and research.”
– Naoto Usuyama, Principal Researcher, Microsoft Research Health Futures
-
Microsoft research copilot experience Summarize how GigaPath is approaching cancer pathology.
Transcript: Lightning Talk 4
GigaPath: Foundation model for digital pathology
Naoto Usuyama, Principal Researcher, Microsoft Research Health Futures
Naoto Usuyama proposes GigaPath, a novel approach for training large vision transformers for gigapixel pathology images, utilizing a diverse real-world cancer patient dataset, with the goal of laying a foundation for cancer pathology AI.
Microsoft Research Forum, March 5, 2024
NAOTO USUYAMA: Hi, my name is Naoto. I’m from Microsoft Health Futures. I’m excited to talk about GigaPath.
Unfortunately, almost everyone gets cancer at some point. And when cancer is suspected, a small portion is taken from a patient, and this small portion is sent to a pathology lab. The pathology lab prepares a sample and creates a pathology slide. And then the pathology slide is examined under a microscope. And this microscopic view provides lots of information into cancer characteristics, profiles, and this information is essential for choosing the best treatment for each patient.
One notable example is immunotherapy. Immunotherapy is, like, one of the cutting-edge cancer treatments, and it works by using a patient’s own immune system, and it’s, like, a new hope for cancer patients. But unfortunately, it doesn’t work for everyone. The key is the tumor microenvironment. Tumor microenvironment means a complex ecosystem within and around the tumor. This includes not just the cancer cells but also normal cells, like immune cells and blood vessels, and how they interact with each other affects the immunotherapy and the success rate. So modeling the pathology images and modeling the tumor microenvironment is very critical.
My slide is not working. OK, thank you …
The pathology image is super detailed, and the size is huge; one file can be a couple gigabytes. And this pathology slide, I’m not sure if you know, but it’s very tiny, only a few centimeters. But with a microscope, you get very high-resolution images, and it can be 120,000 pixels in just one slide. And this size can blow up transformers easily. Typically, vision transformers use only a few hundred tokens, but for us, we get 56 million, so a few hundred tokens and 56 million. And even if we use a larger patch size, we get a lot of tokens. So it is quite challenging to model pathology slide images. So how do we do this?
We are investigating scalable architectures, and one example is LongNet. We are collaborating with Microsoft Research Asia, and the key idea is dilated attention. This dilated attention uses sparse attention patterns instead of dense attention in vanilla transformers. And also, we segment the sequence into smaller blocks and then focus attention within this smaller segment. So sparsity and segmentation make it much more scalable. And we are testing this LongNet idea for pathology images, and that’s the modeling side. And data is critical for foundation models, of course, and we are working with Providence Hospital. Providence Hospital is one of the largest nonprofit hospitals in the US, and together, we are working on creating a large-scale, real-world patient dataset. Our dataset includes more than 1 million cancer patient records. This includes all the clinical notes so text, as well, and genomics data, as well, and radiology images and reports, and, of course, the pathology images. So this is very large scale but also multimodal, longitudinal. So this rich dataset enables us to train a large-scale foundation model. And to make the most of the data, we are exploring self-supervised learning approaches in many ways, like unimodal, multimodal, longitudinal, and that’s basically the GigaPath project: to make the real-world foundation model using the Providence Hospital data.
This project is not possible without many, many collaborators, and we are just scratching the surface, so I’m very excited, and I really hope we can unlock the full potential of the real-world patient data and advance AI for cancer care and research.
Thank you.
Related resources
- Research Lab Microsoft Health Futures