Hosted by Bonnie Kruft, with Rianne van den Berg, Tian Xie, Tristan Naumann, Kristen Severson, and Alex Lu at Microsoft Research Forum, March 2024
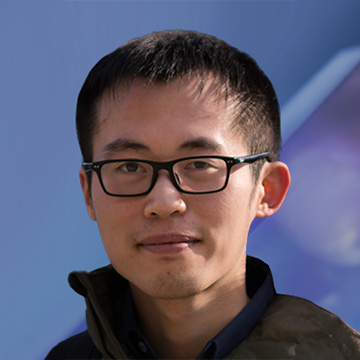
“Just as in the fields of health and biology, machine learning is really beginning to disrupt some of the traditional pipelines that happen in materials discovery.”
– Tian Xie, Principal Research Manager, Microsoft Research AI4Science
-
Microsoft research copilot experience How is AI transforming the natural sciences?
Transcript: Panel Discussion
Transforming the Natural Sciences with AI
Bonnie Kruft, Partner Deputy Director, Microsoft Research AI4Science (Host)
Rianne van den Berg, Principal Research Manager, Microsoft Research AI4Science
Tian Xie, Principal Research Manager, Microsoft Research AI4Science
Tristan Naumann, Principal Researcher, Microsoft Research Health Futures
Kristen Severson, Senior Researcher, Microsoft Research New England
Alex Lu, Senior Researcher, Microsoft Research New England
Microsoft researchers share their advancements in the fields of foundation models, drug discovery, material design, and machine learning. They highlight how deep learning is transforming the natural sciences.
Microsoft Research Forum, March 5, 2024
BONNIE KRUFT: I’m joined here by my colleagues in Microsoft Research. We’re all working in different teams in AI for science, working at the intersection of machine learning and the natural sciences. So my name is Bonnie Kruft. I work in the AI4Science team in Microsoft Research in Cambridge, and I’m joined here with Rianne.
RIANNE VAN DEN BERG: Hi, my name is Rianne van den Berg, and I’m a principal research manager in AI4Science at Microsoft Research located in Amsterdam, in the Netherlands. And I co-lead a project on density functional theory. Yeah, that’s it.
TIAN XIE: Hello, everyone. So my name’s Tian Xie, so I’m a principal research manager at Microsoft Research AI4Science. I’m located in Cambridge in the UK. So I lead the project that works on generative models for materials, so the MatterGen model that Chris has mentioned earlier is coming from our team. So, yeah, very nice to be here.
KRISTEN SEVERSON: Hi, everyone. My name’s Kristen Severson, and I’m a senior researcher in the Bio ML team at Microsoft Research New England. I’m broadly interested in how we can use machine learning for applications in human health with a current focus in computational pathology.
TRISTAN NAUMANN: Hi, everyone. My name is Tristan Naumann. I’m a principal researcher in the Real-world Evidence Group here at Microsoft Research’s Health Futures, where we’re looking to advance health at the speed of AI. My research focus is really at the intersection of artificial intelligence and health care, specifically the application of natural language processing in this space.
ALEX LU: Hi, everyone. My name is Alex Lu. I’m a senior researcher, part of the Bio ML team at Microsoft Research New England. My research concentrates on how we can use AI to make new biological discoveries, particularly in the realm of cellular and molecular biology.
KRUFT: OK, great. So our first question is, what real-world impact have we seen already today in health care, drug discovery, or in materials science?
NAUMANN: Yeah, so maybe I’ll start. I think this is an incredibly exciting time in health care. If we, sort of, think to some of the goals of precision health over the years, it’s really to look to apply the right intervention for the right group of people at the right time. And one of the things that’s crucial to realizing that is really this reality that we need a data-driven learning system that’s able to adapt and then also really incorporate new information instantaneously. Historically, this has been incredibly challenging because much of the data we have in health care is not nicely structured in a clean, easy-to-use way. And so one of the things that’s really incredible about some of these recent advances in generative AI—specifically large language models, LLMs, also large multimodal models—is really this opportunity to have a tool for universal structuring, and unlocking some of that data quickly and efficiently really opens up a lot of new opportunities. I think another thing that’s really nice about some of these techniques in generative AI is the innate accessibility of some of these tools. So a lot of the clinical collaborators, other care staff that we work with, they can use some of these tools, as well. And so really taken together, you have this new opportunity to be able to quickly access a lot of the information that potentially holds the future of medicine.
KRUFT: That’s great. You mentioned universal structuring. Can you touch maybe on an area where that’s used already in health care?
NAUMANN: Yeah. So I think actually maybe a little bit of context. If we think to the cancer space specifically, we have this, sort of, interesting paradigm where the center of care often fails. And so the, sort of, last resort for many patients is a clinical trial. And unfortunately, in the US, very few patients are actually able to enroll in some of these trials. So the number is ranged, but perhaps something like 3 percent. And on the other side, we have a number of pharmaceutical companies who are indicating that there’s actually a really large number of trials that fail because of insufficient number of patients. So up to 40 percent. And so there is this, you know, maybe immediate gap there that we might want to address. And so we’ve looked at some first steps toward this with our partners at Providence health system really looking to start to close up that gap. So specifically, some of the work we did recently was looking at how we could scale clinical trial eligibility criteria. So taking, for example, the unstructured text from something like ClinicalTrials.gov, bringing that into logic formats that could be more easily used by a lot of things downstream. And then really looking to how we can make that accessible to the clinicians who are trying to match that for patients, as well.
KRUFT: That’s great. Thank you. And what about in biology?
LU: Absolutely. I would describe biology as being a very heterogenous and fragmented landscape. I mean, this is not surprising because there are just so many subdisciplines in biology that not everyone uses AI in the exact same way. So, for some context, I concentrate my research on three main areas. I do work in proteins; I do work in omics, which concerns genes and how they’re expressed; and I do work in images, particularly microscopy images. And I would describe each of those fields as basically being at a different level of maturity. So, for example, for proteins, we have very well-founded structure predictors; protein language models are routinely integrated into bioinformatics prediction pipelines. But for omics data, the idea of using a large amount of data to pretrain a model, even though there have been a lot of really inspiring, like, precursors to this work, is really just emerging, and the conversation in that area is just beginning, then. And then similarly for images, which I believe is poised for the next revolution because you can see that there are people, like, really ramping up their data collection efforts and there are these massive datasets that only recently, like, hit the public sphere, but the extent that people have really worked on these datasets compared to the work in proteins is actually very limited, then. So there are a number of factors that influenced this. To speak to a few, one factor is how much a field has, a field’s problems can be posed as well-posed predictable problems for AI. So, for example, in the realm of protein engineering, a lot of these problems can be formalized as well-posed prediction tasks—predict the structure of a protein given the sequence of a protein, for example. But for a lot of things in biology, that’s not necessarily true. The task is more exploratory in nature: “Hey, I’ve got a huge amount of data. Help me understand and comprehend this data.” It’s not really a task that you can easily evaluate whether you’re doing good or not simply because there’s a subjective element to that, then. Another factor is how centralized the dataset sharing and collaboration is. So for example, I’ll point to proteins, again, as a wonderful example of this because for the longest time, even before AI became a thing, it was standard to deposit your protein sequences in a single centralized repository, and then eventually that became the foundation on which many people train their models on now, then. In contrast, I would describe images as almost being, like, the opposite situation, not as if the biologist don’t agree that it’s important to share the data—many do—but the formats, where you share your data, what your data looks like, even, like, your file conventions, all that varies very drastically and so that data has traditionally not been accessible for machine learning practitioners.
KRUFT: Yeah, that’s a great point. Thank you. And what about in materials?
XIE: Yes. So, yeah, similar. Just as in the field of health and in biology, right, machine learning is really beginning to [disrupt] some of the traditional pipelines that happen in materials discovery. So one area that has been especially innovated here was basically there’s this huge area about using large-scale computation, high-throughput screening, to discover new materials. So this was just running large-scale quantum mechanical calculations and to screen thousands, tens of thousands, of materials to find new materials for a variety of different applications. But the limitation of this field was that a lot of these simulations were very, very expensive, so you cannot go into a lot of materials. So one area that Chris mentioned in his keynote was the building up these machine learning emulators. So they have been shown to be able to speed up the simulation of materials properties by at least a thousand times, allowing the community to really screen a lot more materials in a much faster speed than what has been possible before. So another important area is really generative models because for these traditional, like, screening-based methods, I think you are, kind of, limited by the number of candidates, right? You know for materials, so for materials, this is usually in the order of hundreds of thousands of materials that you can screen from. But now with these generative models, you can, kind of, expand into this much larger hypothetical space of at least five to six order magnitude larger by generating materials guided by the property that you’re interested in, like the MatterGen model that we had developed earlier. So this opens up a lot of opportunity for discover much better materials in many different domains. So this research has really created a lot of excitement in the community, across industry, that a lot of these materials companies are beginning to pay much more attention into this AI tools, but obviously this is not as mature as what we have seen in pharma industry, where many of these AI tools has already been integrated into, like, their drug discovery pipelines.
KRUFT: Yeah. So what strategies would you take to increase adoption and trust of those AI models in the materials industry?
XIE: Yeah, I think this is a wonderful question because I think that adoption is really the key for this AI models to have a real-world impact, right. So what I see today in adopting AI models for materials is, kind of, very similar to what we’ve seen in AI for drugs in around maybe 2020. So at that time, there was actually a research coming from MIT where the researchers were … managed to discover a new antibiotic drug from this AI models. So I think this is, kind of, like a wakeup call for a lot of the pharma companies to become paying much more attention into this new AI tools. So after that, we see a lot of investment from the pharma companies to, kind of, developing their internal teams to be able to really utilizing these tools and being able to integrate that into their internal pipelines. So it’s, kind of, a gradual process because it’s also internal trust-building process. At the beginning, a lot of the pharma teams, they don’t trust these models. They would prefer their traditional pipeline, right? But once they see one or two examples, right, of this new drugs designed by this generative models being actually performing better than something that they come up with their traditional pipelines, then they begin to adopt. So I, kind of, see where we are today in the materials as, kind of, similar to this, kind of, early stage where these materials companies begin to try out these models and they are going to be a lot of iteration going forward. But I’m quite optimistic that these AI tools will begin to make a bigger impact in the coming maybe two to three years.
KRUFT: Yeah, thanks, Tian. Having come from the pharmaceutical industry myself, I’ve definitely seen that transformation over the past couple years, so it’ll be really interesting to see that happening in materials, as well. So the next question we have is, AI has been used in different applications in different industries, but how do you think that science is different?
SEVERSON: In the health care space, I think there are two main differences. And the first that I’d want to highlight is data. So Tristan already mentioned this, that a lot of the data in the health space is not in a format such that we could leverage it for AI applications. It’s also often that there are privacy concerns about how we might pool this data together, and it sits siloed in various health systems. So these factors combined means that health data is oftentimes quite small, and that’s a major difference between what we’ve seen in more classic machine learning applications. I do think recently there have been a couple of factors that have started to change this, one being the rise of these large language models that can help us process the data into a format that’s usable as well as just performance gains. I think that those performance gains have inspired the health industry to think, what can we do with these types of models? What types of innovations might we see? The second piece of what differentiates health, though, I would say is an interest in building on prior knowledge. We have a lot of knowledge about diseases and how they manifest, and we don’t want to leave that information on the table when we train machine learning model. So there’s not an interest in using solely black-box approaches but instead building on what’s already known. And we could think of a specific example of that in terms of some of the invariances that were mentioned earlier. So if I focus on a digital pathology image, we have that same rotational invariance where you can rotate the image and it has the same context. One way I think those images vary as compared to natural images is in terms of the resolution. Because of the way the data is generated, we have rather fixed scales, where each pixel maps to a certain number of microns, and we might hope to leverage that information when we’re trying to describe the morphology of the tissue that we’re analyzing. And so while we have that piece at the development side, I think there’s also considerations, how we want to build on that knowledge at the deployment side, where clinicians might be hesitant to leverage something that’s really black box. So how can we build their trust in a similar but different way to what Tian was mentioning?
KRUFT: So tell me, what are some of the key factors for success and trust in those models?
SEVERSON: Yeah, I think there’s a lot of different ways you can use a machine learning model in a health application, but if we focus specifically on the point of care, I think at least in the near term, the gold standard is going to remain randomized controlled trials. An RCT is something that a clinician is already familiar with and is really the standard for evaluating some new tool in the health space. And I think that we know that this is possible in health care. One of our partners in pathology, Paige, actually did go ahead and do an RCT to get FDA approval for their prostate product, which is useful for detecting prostate cancer in tissue samples.
KRUFT: Oh, wow. That’s fascinating.
SEVERSON: And I think one thing that would be interesting to highlight here is some of the different ways the data is generated. So in the health care space, there’s a lot of excitement about leveraging the data that’s already been generated as part of the standard of care. But I think this really varies from some of what I’ve heard about materials, and I’d love for Rianne to talk a little bit more about that.
VAN DEN BERG: Yeah, so I think one of the areas that, or one of the areas where natural sciences and AI, kind of, differentiate itself also from other areas like vision or language or even maybe in health is that the importance of synthetic data should not be underestimated. So, and this goes in particular for, I think, models that are targeting the molecular and the material sciences because, as Chris already mentioned in his presentation, in this case, these models often aim to replace very expensive simulators with faster AI emulators or generators. And so contrary to, for instance, models that are trained for vision or for language, where the data are real images or text that’s scraped off the web, in this case, these models are trained on the data that is generated by the simulators that they aim to replace, right. So we are training on synthetic data, and that has the advantage that we have less privacy concerns, which is a good thing. But there’s also challenges that come with that because generating this data is quite expensive, and there is less publicly available data than in, for instance, areas such as vision and language. So when you want to generate new data, you have to do this with a very effective strategy. So new data should really improve the performance of your model. You shouldn’t just start randomly generating data at large scale without knowing that. And this also highlights, again, the importance that we’ve already mentioned before, that generalizability from small amounts of data is very, very important.
XIE: Yeah, actually, I want to dive a little bit deeper about synthetic data for materials because this is actually really, really important for a lot of machine learning models for materials. Compared with some other spaces, the availability of data that’s coming from experiments is actually quite small for materials. I think mainly because materials are pretty diverse; [there’s a] variety of different class of materials, and the data was pretty sparse in a lot of these domains. So what the community ended up leveraging is basically these large-scale computational simulation workflows that has been developed over the past decade in simulating the materials’ properties of a variety of different material design problems, let’s say the solar cells catalysis or the batteries. So this actually all started from this initiative called Materials Genome Initiative that was established in 2011 by the Obama administration as a way to develop the infrastructure and the simulation capability to use simulation to significantly speed up the materials development process. So building around this initiative … so there has been a lot of effort all around the world in building up this open community over all these workflows to simulate materials and generate data. So this creates a lot of open databases that is currently actually powering the majority of the machine learning models that we have seen today.
KRUFT: So what do you think are the future opportunities for data generation in materials?
XIE: Yeah, I think there are mainly two opportunities going forward that I can see. The first is really the leverage of the new coming cloud infrastructure like what we have here with Azure. So in our team, we have this experience that we were able to utilize a lot of low-priority compute on Azure to really scale up our simulation to generate, to simulate a million materials in just a week, which would normally take, I think, a couple of months if you’re doing this in a more traditional kind of academic setting where you have a server of your group, right; you can only run things in a smaller scale. I think this creates, really creates a lot of opportunity to generating lots of data because you have a lot of compute power available on this cloud infrastructure that is actually underutilized.
KRUFT: Yeah.
XIE: So the second area that I see this creates a lot of opportunity is the autonomous labs that has been gaining to building up in this space of materials, so compared with pharma, where you see a lot of this automation, this is actually a pretty new thing in this space in materials, and in just the in this last one, two years, people has been spending a lot of investment in building out this autonomous labs for a variety of different material design problems, see batteries and also metal-organic framework synthesis, et cetera, and so it used to be that if you were a graduate student, right, it could take maybe one month or several months for you to synthesize a material. But now, with these autonomous labs, you can do tens or even hundreds every month, really significantly speeding up the throughput of experimental data generation. I think this is another very exciting opportunity that I can see in this space of materials for data generation.
KRUFT: Very exciting. And what about in biology, Alex?
LU: Sure. I would say that as a computational biologist, I sometimes feel very fortunate to be working in the field because I don’t struggle with many of the complications with data that my colleagues describe. So, for example, unlike my colleagues in health care, the data did not tend to be private. We’re dealing with microorganisms, basic biology measurements. It’s not associated with patients or human beings, so there’s no issues of showing up, then. And in contrast to my colleagues from materials, in many subdomains of biology, there are scalable data collection processes. So, for example, I remember when I first entered microscopy 10 years ago and people would conceptualize microscopy as, like, this very low-throughput, subjective thing where individual biologists would look at slides under a microscope and that was the extent of understanding data then, right. But since then, we’ve really revolutionized the data collection processes. We now have robot-controlled microscopes, and they can collect tens of thousands—maybe even millions—of images just in the course of a single week. So already you’ve converted like microscopy data into what was originally a low-throughput, qualitative science to a high-throughput and then necessarily a quantitative science, then. And so while I cannot say that every single, like, piece of data collection in biology is scalable at that level, what I can say is that there a lot of efforts to improve the scale at which we collect biology across multiple disciplines. I feel like any domain that I entered, there’s always people thinking about, how do we scale this data collection, then.
But at the same time, just because we have an abundance of data for training doesn’t necessarily mean that we have the right data for training. If you look at what particularly differentiates biology—and, I suspect, by extension a lot of other scientific disciplines—is the whole point is to try to discover something new, right? So by definition that new thing is not going to be captured in your original distribution of data. So take proteins as an example. The most interesting application could be trying to design a protein that has some kind of a property or function that is not seen in any existing protein out there on the planet, then. And so while we have a very large database of proteins—UniProt contains 200 million proteins—all of those are proteins from natural organisms that do exist out there on the planet, then. So there’s already a bit of a mismatch between the data we have to train and what it is we actually want to do with this application, then. So this means that we have to be careful and intentional about the fact our data may not actually reflect what it is that we want to do, then. There are multitudes of approaches to this. One, I think, has been more intentional with data collection, which resonates with themes that Rianne talked about, then. I think in the earlier days of AI, we saw, OK, through preexisting biological databases and then people will use these databases to train models. Now you’re seeing more of an exchange between the ML practitioners and the biologists, then. So you’re seeing a lot of biologists thinking, OK, I need to intentionally collect data to allow the thought of AI efforts, then, and then in doing so, they collect more diverse data. So, for example, in the latest microscopy datasets, what they do is that they’ll intentionally collect data from multiple or different sources across, like, the world, knowing that differences between, like, different laboratories and the way that they collect images is a big barrier for AI, then. The other way I think we should be doing this is just being very intentional about the method that will actually produce it, then, because again, the whole goal is, like, to generalize from that known distribution, to extrapolate beyond that known distribution, to something unknown, then. And I think you have to think from first principles, like what methods are suited to do that extrapolation and what methods are better in distribution.
KRUFT: Yeah, great, great point. So how are you guys—what are some specific techniques that you’re using to create a big impact in science overall?
VAN DEN BERG: So I think that here is worth focusing on something that Alex has mentioned before, and that is the importance of scientific discovery when you look at AI for the natural sciences. And here we can think about scientific discovery in materials and in drugs. And obviously generative models play a very big role here, right, because they can learn what existing materials and drugs look like and use that knowledge to essentially search in a space of unknown materials and drugs. And one particular class of generative models that I’m very excited about and that’s becoming increasingly popular is that of diffusion models and score-based generative models. And these models have been super successful already, for instance, in high-resolution image generation and video. And I think they’re also very naturally suited to target scientific discovery. So the way that they are trained is that at training time, they get data that is corrupted to various degrees, and the model then has the task to try to de-corrupt those data, these corrupted data points in an iterative process. And then at test time, what you can do is you can feed it a data point that is complete noise and iteratively apply this model such that it turns that noisy sample into a sample, for instance, for a new material or a drug. And what is really exciting is that we’ve seen some very cool applications of these type of models already. For instance, in protein sequence generation, something that Alex has already worked on.
LU: Sure, I can speak to that. So this is the EvoDiff work, and the goal of that is to generate novel protein sequences. So to give you some context for this, proteins essentially actualize the majority of biological functions at the molecular level. So to be able to design them has vast application. So, for example, some of the things that people have done with protein design in the past will be to design proteins that can metabolize and break down plastics or to design proteins that can synthesize new, like, pharmaceuticals, then. Obviously, very impactful applications. And frequently, the goal, again, is to try to do something that’s not really present in that of nature. So you really want a protein that can synthesize a new pharmaceutical as opposed to just replicate, like, a protein that already synthesizes, like, a compound that [already can be produced]. So what makes this problem really difficult is just how vastly expansive the search space it. Proteins are made out of building blocks called amino acids. There are 20 different amino acids, then, and then they connect in a chain to form a protein, then. So typically proteins are around a few hundred to a few thousand amino acids in length, then. So when you talk about the search space, it is actually 20 to the x, where x is a few hundred to a few thousand letters, then, and you can see that the strategy for such search space is not really that functional. If you look at the landscape of any possible proteins, the majority of combinations essentially produce just gibberish. You know, not going to, like, produce a viable protein, then. So the goal is to be able to hone into, like, to toe that fine balance between novelty and function. You want to discover novel proteins, but you want to ensure that these proteins are functional in nature, then. So our strategy, yeah, is exactly to use, like, these diffusion models. So this is essentially a discrete diffusion task. Like, you’re trying to predict, OK, each position, what amino acid should be slotted into that particular position, then. And our strategy is essentially to use the distribution of known proteins. I alluded to the UniProt database, which contains, like, natural proteins across all walks of life, then, train on that, then, and extrapolate to generate novel proteins that are hopefully within that functional distribution because, like, since these are proteins from natural organisms, by evolution, the likelihood you, like, the vast majority will be functional, then, but that helps us train a search space so that we design mostly a functional protein, then.
VAN DEN BERG: So I think that ties in really nicely, one of the things you said, to why diffusion models are, I think, very attractive for the natural sciences—because they are, it’s very easy to adapt them to the different types of data that you find or discovery tasks that you have in the natural sciences. So Alex already mentioned that, you know, you can use diffusion models for protein sequence generation, which is inherently discrete data task. But if you were interested in structure generation of proteins, then what you’d need to generate are samples where you describe the positions of the different atoms in the protein, and that’s an inherently continuous problem, right. You have to describe where the positions are of the atoms. Now if you want to take it even a step further and look at, for instance, crystal structure prediction, then you have to describe the generation of an object that has combinations of discrete parts, but also continuous parts, so the positions of the atoms, but you also have to describe what the different types of the atoms are. And I think what’s nice is that diffusion models can very naturally handle all of these scenarios easily. And Tian has actually done some really exciting work recently on this. So maybe you can tell a little bit more about that.
XIE: Yeah, absolutely. So, yeah, I want to talk a little bit more about the MatterGen, which was the model that Chris has also mentioned earlier in his keynote. I think this is such a great example to demonstrate the kind of flexibility of it [diffusion models] being able to handle different kind of types of data—discrete and continuous. So for materials, we usually represent a structure using its so-called unit cell, which is the smallest—because it’s actually an infinite periodic structure, so you want to looking at its smallest repeating unit. So then there are three different attributes. One is the atom types, and second is the atom positions, and last is lattice, which, kind of, defines this periodic box and defines how this crystal structure repeats, right, in this three-dimensional space. So the atom type is a discrete variable, and atom position and lattice, they are continuous variables, and they are all geometry. For example, coordinates are in this periodic space where the lattice, the, kind of … you need to impose some constraints to avoid that getting into a pretty skewed lattice. So due to the flexibility of the diffusion model, so we actually build a very specialized diffusion process for each one of these attributes—atom types, positions, and lattice. And by combining together, this, kind of, lead into this MatterGen model that we have found that by doing these innovations around how do you build diffusion for different attributes, we’re able to significantly outperform traditional previous models that takes, that does it in a less careful way. So I think another advantage of diffusion model is really the ability to add in different conditions so that you can guide the generation towards the direction that you’re interested in. So I think most of you are probably familiar with this model, like DALL-E 2, DALL-E 3, right, that is text-based generative model that you can, kind of, guide the generation of an image using these text values. So in materials, we do something very similar. We can actually guide the material generation using things like electronic property, magnetic property, or even things like chemistry. And the flexibility of diffusion models basically allow us to very easily to add these different conditions into the model. This actually provides a much more flexible tool for the materials science community to basically being able to compose different types of constraints that they are interested in to solve in their own material design problems.
NAUMANN: Yeah, and maybe, maybe I’ll touch a little bit on two of the models that our group has worked on recently in collaboration with the Deep Learning Group and collaborators in health and life sciences. One of them based in diffusion; the other not as much. So we have some variety in this space, as well. The first is this model BiomedJourney, where we’re actually looking at this task of counterfactual image generation. So you can, sort of, imagine taking a medical image, maybe something like a chest X-ray, adding some sort of text like maybe “resolved pleural effusion” or some other condition that you’re hoping to apply, and then trying to see, you know, what would that look like. And you can imagine a variety of potential use cases, whether it’s, sort of, training or even synthetic generation of data for health care cases. And in another one of the works, LLaVA-Med, we’re really looking at, you know, how can we create, sort of, a GPT-like interface that’s able to use both images and, and some of the text data, as well, and interact with some of those images in a much more useful way for some of the clinical practitioners that we work with. I think actually touching on one of the things that Kristen had mentioned earlier, you know, there is always this desire to use some of the data that’s actually out there. And so in this latter work, one of the things that we focused on was really also the creation of a large dataset gathered from publicly available data sources in order to help both support this model and then also help support other similar models in this space. And I think one of the things, Tian, that you had mentioned, as well, is there is this broader community. And so I think one of the, sort of, interesting things that, sort of, come out here is I think we’re all working, you know, on of course some interesting things but also to support the broader intersectional communities at this space, as well.
KRUFT: Yeah. So we can really see the transformative power of generative AI towards the discovery of new medicines, in materials, or even in health care, too. So finally, I want to touch on collaborations. So as machine learning researchers, it’s really important for you to optimize your collaborations with domain experts. So, for example, in my team, we have a close collaboration with Novartis to train new AI models to help us discover new small-molecule drug candidates. But I’m curious. What collaborations do you have now, and how are you optimizing that collaboration with the domain expert?
SEVERSON: Yeah, we have several different collaborators in the pathology space in addition to Paige that I mentioned earlier, who’s an AI software company. We’re also working with Volastra, who’s a therapeutics company, and Providence health system. And I think the motivation for having this—oh, and that’s not even an exhaustive list of our pathology partners. So I think the motivation for having this diverse set is to make sure that we really understand the different ways these tools could be applied. It gives us a diversity of perspective that then allows us to think about where could we have the biggest impact, like where is AI going to make a difference, and bring all those perspectives together to form our research strategy and ideally build something that can cut across these different applications of point-of-care diagnostics and therapeutic discovery.
KRUFT: That’s great.
NAUMANN: Yeah. This is an incredible, incredibly important set of points because I think a lot of the collaborators we work with actually, sort of, ground the reality that our work resides in. And you mentioned Providence health systems, and, sort of, going back to one of the things I mentioned earlier around clinical trial matching and, sort of, the importance in that space, that was, you know, primarily a project that was, sort of, born of a desire to, sort of, be able to do more than we can currently in this space. And similar to some of the registry work that we’ve done with them, as well. And so I think mostly just to say, yeah, I think this is … our collaborators across a variety of these projects really help us make sure that we’re having the impact that we’re looking for.
KRUFT: I’m curious about internal collaborations. Rianne, Tian, any thoughts about that?
VAN DEN BERG: Yeah, so we work on a project on density functional theory, which is a method that we can use to do quantum calculations, which are relevant for chemistry and physics and materials science, and our team is super interdisciplinary. We have researchers in machine learning but also researchers in quantum physics and chemistry. And although, I have to say, of course, in the beginning when you set up a project like that, I would say interdisciplinary communication can be a little bit of a challenge because you have to learn how to speak each other’s language. But after, kind of, going through that process, it definitely gets better over time. And after doing that now for about a year, I’m 100 percent convinced that if you don’t have such intense daily collaborations, it’s, I think, impossible to have success in some of these projects because we really need to have a really in-depth understanding of what the problem is that we’re trying to solve, also have some sense of what the historical developments were before AI tried to come in and solve this problem, and what’s really needed to make a breakthrough that, you know, the scientific community that has been working on this project, on this type of problem, before AI came in, would also perceive as a suitable breakthrough. So yeah …
XIE: Yeah, I just want to say I 100 percent agree with what you have just said because it’s a very similar situation in our team. So our team, we have people from machine learning background and also from materials science background. There are also people who do a little bit of a computation; there are people who are coming more from the experimental side. So I think it really takes quite a while for the entire team to be able to, kind of, understand each other’s language, right, and be able to speak in the same language that allows for this kind of interdisciplinary communication. I find this to be very, very beneficial because for the machine … for the people from the machine learning background, right, so it’s very important to be able to contextualize your result, right, in the language of scientific domain to understand, is this a trivial result, or is this a result that is actually meaningful, right? Would that actually change how materials scientists design new materials, right? So on the other hand, it’s also very important for the domain scientist to be able to speak the language of machine learning because it takes quite a lot of effort to be able to translate your domain knowledge in a language that a machine learning researcher can understand—for example, how to build up the proper evaluation pipeline, right. How to translate [your scientific intuition into a single scaler number that allows] faster iteration of model development. I think all of this is not possible without interdisciplinary communication and not even within our team. I would say even broader in this community, right, of AI for science community. I have seen a major, a lot of improvement just in the last two years that people from the machine learning research groups and people from the domain research groups really begin to talk to each other, right, a lot of these open workshops. And I think that’s really the key for the success of applying AI to scientific domains.
KRUFT: Yeah, wonderful. Well, thanks everyone. I want to wrap up by just extending my deepest gratitude to my esteemed colleagues here and especially for their pioneering work in the AI for science domain. And thank you to everyone for joining us and listening to us today. So stay tuned. We have some research lightning talks coming up next. Thank you.
Related resources
- Research Lab Microsoft Research AI for Science
- Research Lab Microsoft Research Lab – New England
- Research Lab Microsoft Health Futures