About
For the most up-to-date information, please visit my personal website (opens in new tab).
I am a Principal Researcher Manager in the AI Frontiers lab (opens in new tab) of Microsoft Research, where I lead our work on Large Action Models (LAMs). Our goal is to build action-oriented, multimodal transformer (and beyond) models that can act like humans (and beyond) in domains such as web/OS interaction, car racing, and gaming. Previously I was part of the Microsoft Research New York City (opens in new tab) lab, who we share a building with and continue to work closely together; before that, I was a researcher in the MSR Silicon Valley (opens in new tab) lab. My research trajectory started with distributed systems and data structures, evolved to incorporate machine learning, and is currently most inspired by human behavior and improvement. The Maia project (opens in new tab) is an example of this perspective.
I received my PhD from the Department of Computer Science at Princeton University, where I worked with Robert Tarjan (opens in new tab) in the theory group and Michael Freedman (opens in new tab) in the systems group. I spent my final year as a junior research scientist at New York University working with Jinyang Li (opens in new tab). Prior to my PhD, I worked for three years in the Network Load Balancing group of Windows Server at Microsoft. I received my S.B. and M.Eng. in Computer Science from MIT, where my M. Eng thesis advisor was Charles Leiserson (opens in new tab).
Microsoft Research highlights
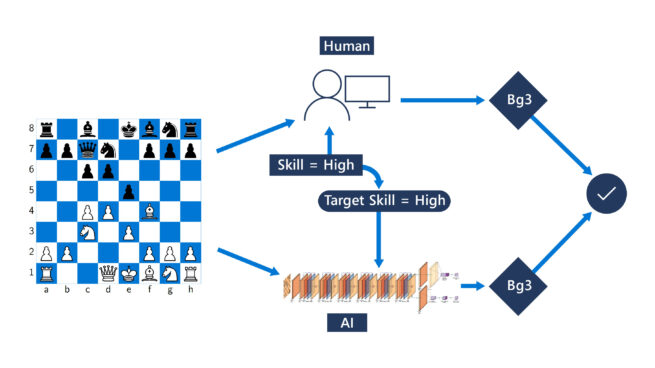
The human side of AI for chess
As artificial intelligence continues its rapid progress, equaling or surpassing human performance on benchmarks in an increasing range of tasks, researchers in the field are directing more effort to the interaction between humans and AI in domains where both are…
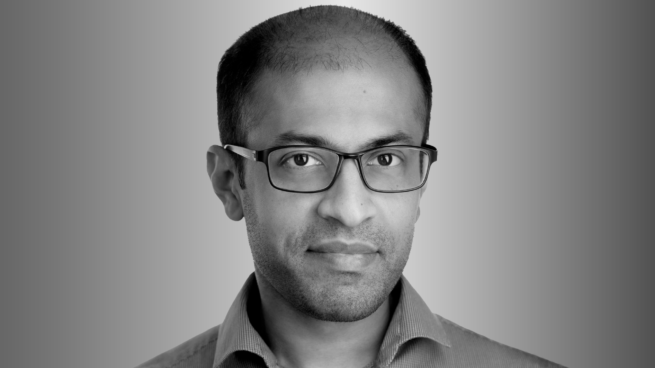
Harvesting randomness, HAIbrid algorithms and safe AI with Dr. Siddhartha Sen
Episode 116 | May 27, 2020 - Dr. Siddhartha Sen is a Principal Researcher in MSR’s New York City lab, and his research interests are, if not impossible, at least impossible sounding: optimal decision making, universal data structures, and verifiably safe AI. On the podcast, he tells us how he’s using reinforcement learning and HAIbrid algorithms to tap the best of both human and machine intelligence and develop AI that’s minimally disruptive, synergistic with human solutions, and safe.