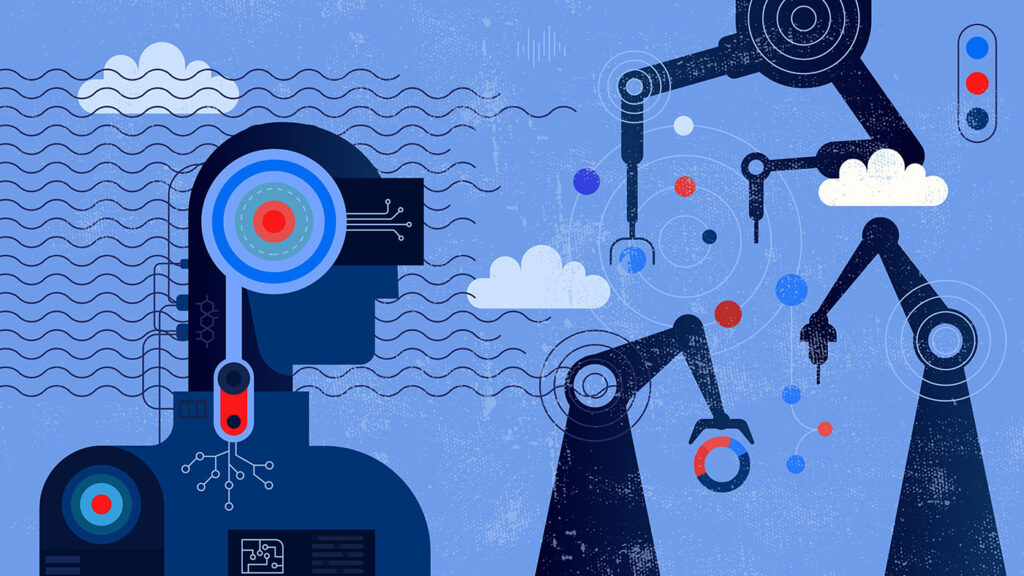
Harnessing the power of artificial intelligence (AI) to revolutionize networking, creating intelligent systems that optimize performance, adapt to dynamic environments, and enhance user experiences.
Related projects
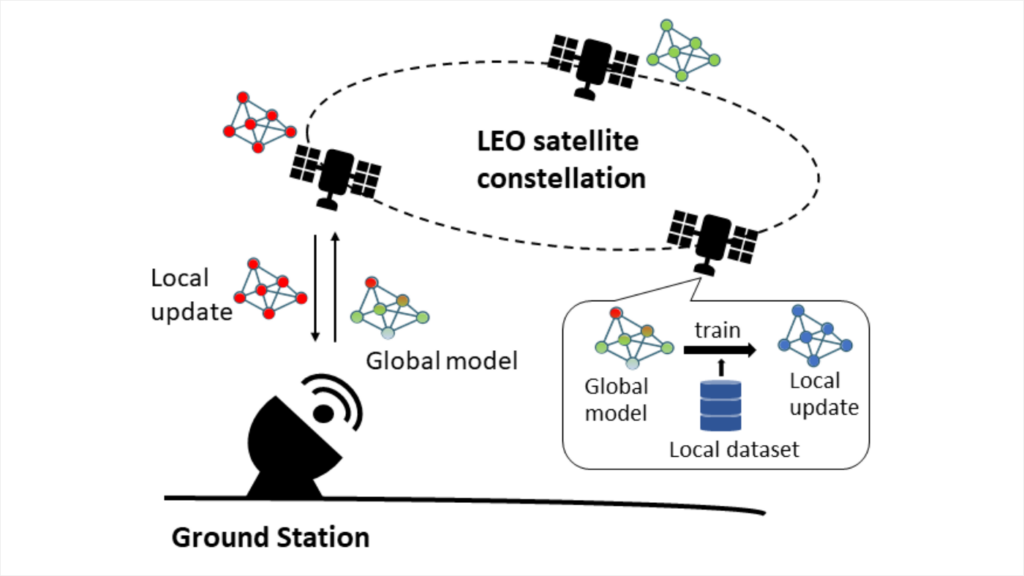
Artificial intelligence training in space
Large-scale deployments of low Earth orbit satellites collect massive amount of Earth imageries and sensor data, but it is increasingly infeasible to download all the high-resolution images and train the corresponding AI models on the ground. In this project, we focus on novel distributed and federated learning frameworks (opens in new tab) that enables ground stations and satellites collaboratively train AI models without downloading all the data to the ground.
Related vertical(s): Edge computing
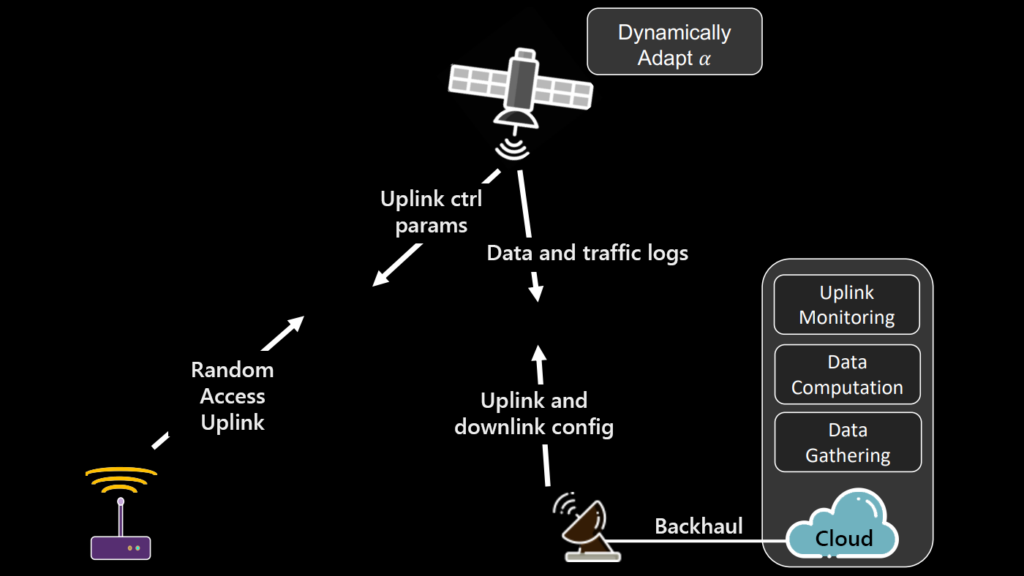
Cloudification of network stack for IoT in space
The “new space” revolution is bringing about a new era of innovation and possibility in the industry as private companies are pushing the boundaries of what is possible by launching thousands of Low Earth Orbit (LEO) satellites. One of the most exciting developments is the use of small IoT picosatellites (picosats) that envisions offering a cost-effective way to connect millions of IoT devices around the world that may not have access to terrestrial networks. The small size and low-complexity hardware of these satellites make them easy to build and launch into orbit, resulting in rapid scaling of IoT picosat networks. As picosat constellations scale, their traditional networking stack becomes the bottleneck because it does not incorporate constraints specific to picosat constellations. In this project, we innovate a new end-to-end networking stack for IoT in space by introducing cloudification of the network stack.
Related vertical(s): IoT
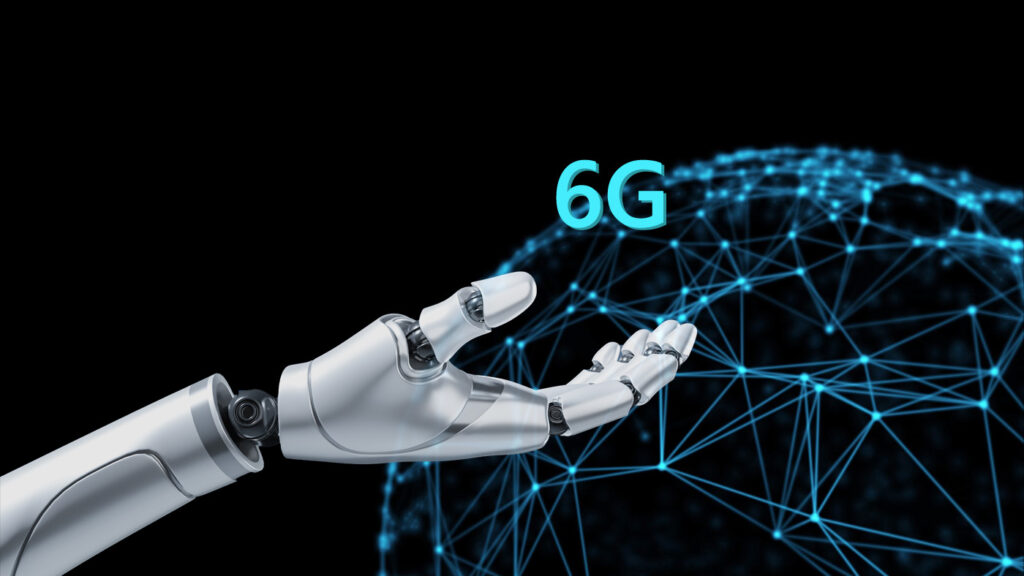
Leveraging AI for Pruning Control Overhead in 5G/6G
Networks In this project, we are leveraging AI and data driven tools to develop technologies that can help reduce the control overheads in 5G and future 6G cellular networks. The project tackles both header information encoded in data packets at different layers of the network stack, as well as control messages that are exchanged between the user device and the cellular RAN and core to manage functions like device registration and handoffs. Traditional network protocol design has maintained a logical separation between the user plane and the control plane. However, this project aims to break this separation and leverages correlated traffic and user patterns from the data plane to implicitly infer control plane events without the need to explicitly transmit control messages on the network. This would help improve network performance in terms of throughput and latency and would allow us to handle control events like handoffs proactively rather than reactively.
Related vertical(s): none