Academic research plays such an important role in advancing science, technology, culture, and society. This grant program helps ensure this community has access to the latest and leading AI models.
Brad Smith, Vice Chair and President
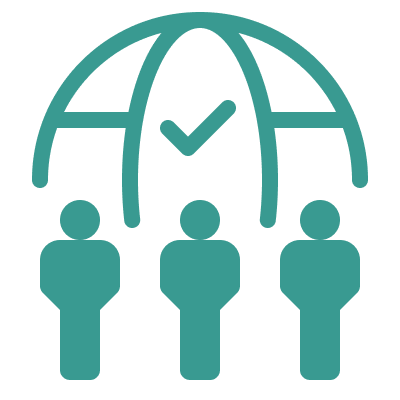
AFMR Goal: Improve human interactions via sociotechnical research
which increases trust, human ingenuity, creativity, and productivity, and decreases the digital divide while reducing the risks of developing AI which does not benefit individuals and society
The research projects primarily focus on enhancing language models, emphasizing underrepresented languages and cultures. Projects aim to improve the accuracy of health-related responses and fine-tune models for specific languages like Vietnamese and various Indian languages. Cultural intelligence is a key goal, promoting linguistic inclusivity and understanding model behavior with knowledge graph tools. Additional efforts involve developing model-editing techniques for interpretability and robustness, particularly in underrepresented languages. The overarching aim is to enhance language models for improved accuracy, adaptability, and inclusivity across diverse languages and cultures.
-
University of Pretoria: Vukosi Marivate (PI)
The goal of this study is to extend the capabilities of foundation models [6] for ESL-speaking African communities by enhancing their ability to understand and generate content that accurately reflects the continentโs socio-cultural specifics. This involves adapting models to usersโ specific needs, and linguistic styles; making the models more accessible and equitable for underrepresented groups. Through localized tuning and feedback, the project seeks to reduce performance gaps and tailor foundation models for diverse uses such as in legal, finance, and agriculture in Africa. The overarching goal is to provide users with the agency to customize foundation (language) models to their unique situations.
-
University of Waterloo: Jimmy Lin (PI)
The proposal aims to build robust foundation models for African languages to bridge the technology gap affecting these communities. The project objectives include design and optimization of model architecture, multilingual transfer learning, evaluation, and making the resources openly available.
Related papers:
-
University of British Columbia: Vered Shwartz (PI)
The proposal aims at addressing the cultural bias in Large Language Models (LLMs), which currently hold a heavy Western, North American, or even US-centric lens. By constructing a new dataset consisting of narratives that evoke social norms, the proposal aims to test the values of English LLMs as they reflect in real-world scenarios and better align the responses of LLMs with the values of diverse cultures.
Related paper:
-
Kennesaw State University: Dylan Goldblatt (PI)
This project aims to explore applications of AI to provide personalized and culturally-responsive support for second language learners at KSU. The objectives are to establish whether an AI learning support approach improves performance and engagement in language courses; if the approach helps narrow the achievement and engagement gap for underprepared students; and whether the support approach is successful across various languages.
-
New York University: Duygu Ataman (PI)
Recent advances have brought Large Language Models (LLMs) to an important stage that will play a significant role in shaping the next generation of applications in essential social domains, such as education and the media. Despite the continuous exploration of its remarkable capabilities, the performance of state-of-the-art models in most languages typically falls short of matching their counterparts in English. This project aims to bridge this gap by developing an adaptation methodology to improve LLM compatibility with under-resourced languages. The study uses Turkic languages as a case study, whose grammatical features present a challenging yet ideal setting for assessing NLP models.
Related papers:
-
Georgia Institute of Technology: Srijan Kumar (PI)
Investigate the capabilities of GPT-4 and its effectiveness in answering health-related queries in various languages. Our research will develop a comprehensive understanding of how broadly applicable the health-related reasoning abilities of foundational models are beyond the English language.
Related papers:
-
IIT Gandhinagar: Mayank Singh (PI)
The proposal plans to develop model-editing techniques that can localize multilingual information and selectively update the parameters of Large Language Models (LLMs). The project involves experimenting with parameter-preserving and parameter-updating editing techniques. The goal of the project is to enhance LLMs in terms of interpretability, robustness, and factual accuracy for diverse communities.
Related papers:
-
IIT Bombay: Soumen Chakrabarti (PI)
Our focus will be on the behavior of the latest generation of LLMs and their interaction with knowledge graph (KG) retrieval tools, in the context of Indian low-resource languages (LRLs), because it is easier to locate users of such languages locally, and code-switched texts.
-
University College London: Pontus Stenetorp (PI)
The proposal aims to improve the performance of Large Language Models (LLMs) on African languages by augmenting low-resource African text data with synthetic data. It proposes a two-step method involving benchmarking tasks to understand the performance gap followed by generating training data for African languages.
Related papers:
-
IIT Kharagpur: Niloy Ganguly (PI)
The proposal plans to analyze the performance of Large Language Models (LLMs) for Indian languages. Despite their proven utility, LLMs have not shown significant improvement for tasks in Indian languages compared to high-resource languages, possibly due to an underrepresented training corpus. The research aims to extensively benchmark LLMs’ capabilities, strengths, and weaknesses for various tasks in Indian languages. The goal is to identify ‘good’, ‘bad’, and ‘ugly’ performance cases and develop strategies for improvement, potentially addressing the underrepresentation of Indian languages in LLM performances.
Related paper:
-
KAIST: Alice Oh (PI)
Develop a culturally-intelligent language model by creating a red-teaming dataset that evaluates actions in different cultures and testing the language modelโs responses in various language and cultural settings. The goal is to improve NLP modelsโ awareness of cultural diversity and their ability to generate culturally intelligent responses.
-
Saarland University: Dietrich Klakow (PI)
The project aims to advance research in multilingual foundation models, focusing on closing the gap in capabilities between English and non-English languages. The team plans to analyze the cross-lingual transfer abilities of foundation models, and seek to enhance these abilities with a focus on in-context learning.
Related papers:
-
IIT Bombay: Soumen Chakrabarti (PI)
We are probing LLMs to detect presence or absence of knowledge in multi-lingual knowledge graphs, with a focus on low resource languages (LRLs). As we move from popular to even slightly obscure entities and relations, we are finding that the coverage and reliability of LLMs fall of perceptibly. Packaging a knowledge query in a prompt context, as well as fair evaluation of text output against structured gold knowledge, are proving challenging. We are also comparing LLMs against pure graph embedding techniques. We are finding that these two families of techniques make uncorrelated errors, suggesting a unified architecture leveraging the strengths of both.
Related papers:
- CRUSH4SQL: Collective Retrieval Using Schema Hallucination For Text2SQL (opens in new tab)
- Frugal LMs Trained to Invoke Symbolic Solvers Achieve Parameter-Efficient Arithmetic Reasoning (opens in new tab)
- Small Language Models Fine-tuned to Coordinate Larger Language Models improve Complex Reasoning (opens in new tab)
-
Ho Chi Minh City University of Technology: Duc Nguyen (PI)
This proposal aims to create a finetuned large language model (LLaMa-2) specifically for Vietnamese using the QLoRa technique. The researchers seek to bring about proficiency in Vietnamese that rivals human-level communication while maintaining the vast knowledge base of the original model. An evaluation against other commercial models is also planned.
Related paper: