Academic research plays such an important role in advancing science, technology, culture, and society. This grant program helps ensure this community has access to the latest and leading AI models.
Brad Smith, Vice Chair and President
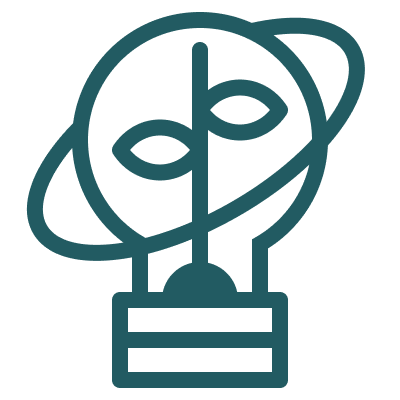
AFMR Goal: Accelerate scientific discovery in natural sciences
via proactive knowledge discovery, hypothesis generation, and multiscale multimodal data generation
These projects focus on using foundation models to enhance knowledge discovery and hypothesis generation across many different areas. They particularly leverage the ability of general models to make sense of the exponentially growing volume of scientific literature in astronomy, materials science, and neuroscience. These efforts include exploring domain-specific prompt engineering and specializing foundation models through fine-tuning using techniques such as Low-Rank Adaption (LoRA). A series of proposals are dedicated to biomedical and life sciences research and innovation, including specialized models for drug discovery, genomics, protein engineering, and rare diseases. These proposals underscore the potential of foundation models to accelerate scientific discovery and innovation across many fields and disciplines.
-
University of Texas at Arlington: Miao Yin (PI)
Ion chromatography (IC) is a powerful analytical chemistry technique for selective, sensitive quantification of aqueous ions spanning applications from environmental monitoring to biopharma pipelines. However, intrinsic slow analysis times severely throttle sample throughput. This project intends to develop an artificial intelligence-based platform accelerating IC by leveraging immense datasets from vast historical runs coupled with large foundation models tailored to effectively encode complex interactive influences of system parameters spanning columns, eluents, and detectors on separation performance into predictive modeling engines on Microsoft Azure. Additionally, a special tuning algorithm with analytical chemistry specialists’ feedback will be developed to ensure the correct prediction of the large foundation IC model. Broader anticipated impacts are poised to revolutionize ion chromatography practices with AI across academic, manufacturing, and innovation areas while providing students at MSI with interdisciplinary research opportunities incorporating computer science and analytical chemistry.
-
Georgia Institute of Technology: Yunan Luo (PI)
This proposal aims to leverage foundation models, including large language models trained on natural language and protein sequences, to advance protein function prediction and optimization. Two key areas of focus are 1) protein function prediction – predicting the biological roles of natural proteins and 2) protein function optimization – predicting which sequence mutations are beneficial for enhancing the function of natural proteins.
Related papers:
- Calibrated geometric deep learning improves kinase–drug binding predictions (opens in new tab)
- Leveraging conformal prediction to annotate enzyme function space with limited false positives (opens in new tab)
- Machine learning-guided co-optimization of fitness and diversity facilitates combinatorial library design in enzyme engineering (opens in new tab)
-
University College London: Bradley Love (PI)
The project intends to utilize large language models (LLMs) to aid in the accumulation and assimilation of vast scientific literatures, especially in the field of neuroscience. The proposal aims to create BrainGPT, an AI tool for navigating and understanding large pools of data. The model will generate data patterns based on the scientific literature, assist in identifying anomalous findings, and offer insights for novel study designs. Additionally, the team intends to open source the models and training data for scientific scrutiny and improvements, fostering participation from the scientific community.
Related papers:
-
New Mexico State University: Huiping Cao (PI)
Data-driven machine learning (ML) models built on large amounts of data have gained great success in many applications. However, their success is less observed in scientific domains. Scientific discoveries and hypothesis generation largely depends on knowledge (commonsense knowledge and expert-domain knowledge). Most of such knowledge is scattered in different sources and such knowledge is rarely utilized in data-driven ML models. Developing ML models that can take both data and knowledge as input in the learning process is still in its infancy.
Many scientific domains collect multi-modality data. However, there is no good benchmark multi-modal datasets to evaluate foundation models.
This project will design and develop novel neural network models to extract domain knowledge, incorporate domain knowledge and account for multi-modality data in the learning framework to improve learning accuracy and efficiency. The proposed methods will be applied to one scientific domain, animal sciences, to validate their usefulness, and generate knowledge base and a multi-modality datasets as a benchmark dataset.
-
University of California, Los Angeles: Aditya Grover (PI)
The project proposes to develop a few-shot machine learning model to learn and optimize multi-task deep learning surrogates across various scientific and engineering domains. The plan includes unsupervised pretraining on large unlabelled datasets, followed by fine-tuning and evaluation on multiple disciplines, including bioengineering, material science, and mechanical design.
-
Harvard University: Alyssa Goodman (PI)
We aim to enhance human interaction with astronomy literature by utilizing the capabilities of the Large Language Models, particularly GPT-4. We employ in-context prompting techniques to expose the model to astronomy papers to build an astronomy-focused chat application to engage the broader community. On the research track, we want to explore the potential foundation models have to generate novel scientific hypotheses. Specifically, we use GPT-4 to construct an instruction set of scientific ideas to fine-tune smaller models on this astronomy-specific downstream task. To assess their output’s accuracy, feasibility and creativity, we employ a hybrid evaluation strategy consisting of human experts and judge GPT-4 instances. Our research will illuminate a novel and unique way of applying LLMs in the scientific arena.
Related papers:
-
University of Toronto Scarborough: Oleksandr Voznyy (PI)
The proposal aims to establish Large Language Model (LLM) agents for inorganic materials discovery by augmenting GPT-3.5 with external tools and databases. The team will develop new text representations for the 3D structures of inorganic materials in order to enable discovery of materials for applications like catalysts, batteries, and photovoltaics.
-
Imperial College London: Aaron Zhao (PI)
The proposal aims to enhance the understanding of complex genomic data by developing a novel Machine Learning framework. Through the use of a new mathematical formulation termed ‘hybrid graphs’, it is suggested that gene expression prediction can be improved beyond the capabilities of current sequence-based approaches. The proposal is also set to construct new databases and theoretical frameworks geared towards genomic data, addressing a current gap in the field.
Related papers:
-
University of Washington: Georg Seelig (PI)
This proposal aims to develop a protein document dataset based on ontology and interaction annotations that will be used for developing protein language models (pLMs) capable of handling multi-protein inputs without linker strings. To take advantage of a structured protein training set, loss and training techniques inspired by natural language models such as RoBERTa will be used. To evaluate if the dataset and training approach generates more informative embeddings, we will evaluate using embeddings for tasks like functional prediction and low-N protein modeling.
-
University of New South Wales: Imran Razzak(PI)
This research leverages Foundation Models to generate structured knowledge from materials science literature. Goals include enhancement of pre-existing datasets, making data in material science literature more discoverable, interoperable, and reusable, and simplifying the data mining workflow in materials science. The approach includes dataset management and construction, information extraction and inference, and knowledge discovery.
Related paper:
-
Yale University: Arman Cohan (PI)
The proposal focuses on making connections within scholarly documents using AI to accelerate scientific discovery. It aims to develop NLP systems that can generate reliable and trustworthy long-form summaries in response to user queries. The ultimate goal is to make it easier for users to comprehend vast amounts of scientific literature and foster faster scientific exploration.
Related papers:
- DocMath-Eval: Evaluating Math Reasoning Capabilities of LLMs in Understanding Long and Specialized Documents (opens in new tab)
- FinanceMath: Knowledge-Intensive Math Reasoning in Finance Domains (opens in new tab)
- On Evaluating the Integration of Reasoning and Action in LLM Agents with Database Question Answering (opens in new tab)
- TAPERA: Enhancing Faithfulness and Interpretability in Long-Form Table QA by Content Planning and Execution-based Reasoning (opens in new tab)
-
Carnegie Mellon University: Larry Pileggi (PI)
The proposal presents a new approach to Situation Awareness based on a Physics-ML synergy approach for which both the physical and ML models are embedded throughout the process to augment each other. This synergy framework enables fast, accurate, and end-to-end situation awareness that integrates system identification, anomaly detection and root cause diagnosis capabilities. The approach incorporates state of the art ML into the operation pipeline of real systems toward advanced operational efficiency, security, and reliable automatic control decision-making.
-
University of California, San Francisco: Tanja Kortemme (PI)
This proposal aims to train a foundation model, Frame2seq, for protein sequence design. Frame2seq is a structure-conditioned masked language model with state-of-the-art accuracy and speed. Frame2seq will accelerate design of new functional proteins by robustly sampling sequence space unexplored in nature. This research has broad applications in material science, biotechnology, synthetic biology, and medicine.
-
University of Illinois Urbana-Champaign: Haohan Wang (PI)
This proposal aims to develop a Team of AI-made Scientists (TAIS) that could dissect complex research questions, pull knowledge from a vast array of academic literature and databases, and employ quantitative and qualitative analysis to uncover deeper insights. The project tackles two main points: statistical trustworthiness (developing mathematical principles and parameter-efficient learning frameworks for large models) and collaborative trustworthiness (formulating an interactive paradigm for large models to work together).
Related papers:
- GenoTEX: A Benchmark for Evaluating LLM-Based Exploration of Gene Expression Data in Alignment with Bioinformaticians (opens in new tab)
- GUARD: Role-playing to Generate Natural-language Jailbreakings to Test Guideline Adherence of Large Language Models (opens in new tab)
- Jailbreaking Large Language Models Against Moderation Guardrails via Cipher Characters (opens in new tab)
- Toward a Team of AI-made Scientists for Scientific Discovery from Gene Expression Data (opens in new tab)
-
The Ohio State University: Yuan-Sen Ting (PI)
The proposal aims to adapt Large Language Models (LLMs) to address complex research queries within the field of astronomy, where current general-purpose LLMs often fall short. The team proposes to develop Foundation models adapted for astronomical research, using over 300,000 LaTeX papers and employing GPT-4-generated instructions for precision fine-tuning. The resulting model will be used for conversational question-and-answering (QA) and hypothesis-generation tasks.
Related papers:
- AstroLLaMA-Chat: Scaling AstroLLaMA with Conversational and Diverse Datasets (opens in new tab)
- AstroMLab 2: AstroLLaMA-2-70B Model and Benchmarking Specialised LLMs for Astronomy (opens in new tab)
- AstroMLab 3: Achieving GPT-4o Level Performance in Astronomy with a Specialized 8B-Parameter Large Language Model (opens in new tab)
- Interpreting Multi-band Galaxy Observations with Large Language Model-Based Agents (opens in new tab)
- Knowledge Graph in Astronomical Research with Large Language Models: Quantifying Driving Forces in Interdisciplinary Scientific Discovery (opens in new tab)
- pathfinder: A Semantic Framework for Literature Review and Knowledge Discovery in Astronomy (opens in new tab)
-
University of New South Wales: Bram Hoex (PI)
The proposal emphasizes using unsupervised word embeddings for predicting functional materials through a comprehensive assessment of various embedding methodologies and foundational models. The research framework uses language models for scientific discovery and analysis of the latent knowledge in publications.
Related paper:
-
Université de Montréal: Glen Berseth (PI)
The proposed research project aims to explore how large language models (LLMs) can assist in reducing the search space over molecular design. The researchers plan to formulate the molecular search problem as a sequence-generation problem, and develop an approach that leverages text-based RL to enhance molecular discovery efforts. Proposed methods include improving the objectives of research with more grounded metrics for evaluation and enhancing generalization by curating and fine-tuning datasets from related design problems.
-
University of Washington: Sheng Wang (PI)
We propose to develop GPT-BLIAM, a model that utilizes GPT models to generate sentence descriptions for diseases, proteins, and their interactions, to enable the prediction of protein-disease associations. Our team will evaluate the model using existing protein-disease association databases and incorporate domain knowledge from Human Phenotype Ontology to learn prompts for rare diseases. Our goal is to improve the quality of the protein and disease embeddings and develop a machine learning model that can predict unknown protein-disease associations.