News & features
![An illustration of the KEAR architecture represented by five panels side by side. The first contains an input question—“What is a treat that your dog will enjoy?”—and the answer choices “salad,” “petted,” “affection,” “bone,” and “lots of attention.” The second panel has three boxes, each representing retrieval from a specific knowledge source. A box labeled “Knowledge Graph” has a silhouette of a dog and underneath it and labeled “desires” a silhouette of a dog being petted; a heart representing “affection”; a bone; and clapping hands representing “lots of attention.” A box labeled “relevant questions” has the question “What do dogs like to eat?” and the accompanying answer “Bones.” A boxed labeled “dictionary” contains the definition of “bone”: “a composite material making up the skeleton of most vertebrates.” The third panel, labeled “concatenation with input,” contains the input question followed by “Dog, desires, bone. Dog, desires, lots of attention” followed by the relevant question and finally the dictionary definition of bone. In between each is a separation token [SEP]. The fourth panel is labeled “language model” and contains a quote box labeled “language services,” a cube labeled “model,” and left and right braces punctuation within a circle labeled “language understanding.” The fifth panel is labeled “output” and includes silhouettes of each of the five answer choices. The silhouette of the bone is highlighted in blue, representing the appropriate response.](https://www.microsoft.com/en-us/research/uploads/prod/2021/12/1400x788_Common_Sense_still_no_logo-1-480x280.jpg)
Azure AI milestone: Microsoft KEAR surpasses human performance on CommonsenseQA benchmark
| Yichong Xu, Chenguang Zhu, Shuohang Wang, Michael Zeng, and Xuedong Huang
KEAR (Knowledgeable External Attention for commonsense Reasoning)—along with recent milestones in computer vision and neural text-to-speech—is part of a larger Azure AI mission to provide relevant, meaningful AI solutions and services that work better for people because they better capture how people learn and…
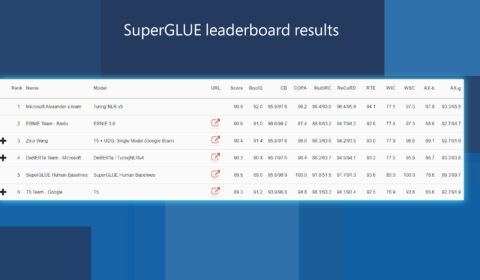
Efficiently and effectively scaling up language model pretraining for best language representation model on GLUE and SuperGLUE
| Jianfeng Gao and Saurabh Tiwary
As part of Microsoft AI at Scale (opens in new tab), the Turing family of NLP models are being used at scale across Microsoft to enable the next generation of AI experiences. Today, we are happy to announce that the…

Speller100: Zero-shot spelling correction at scale for 100-plus languages
| Jingwen Lu, Jidong Long (龙继东), and Rangan Majumder
At Microsoft Bing, our mission is to delight users everywhere with the best search experience. We serve a diverse set of customers all over the planet who issue queries in over 100 languages. In search we’ve found about 15% of…

Microsoft DeBERTa surpasses human performance on the SuperGLUE benchmark
| Pengcheng He, Xiaodong Liu, Jianfeng Gao, and Weizhu Chen
Natural language understanding (NLU) is one of the longest running goals in AI, and SuperGLUE is currently among the most challenging benchmarks for evaluating NLU models. The benchmark consists of a wide range of NLU tasks, including question answering, natural…
In the news | VentureBeat
AI models from Microsoft and Google already surpass human performance on the SuperGLUE language benchmark
When SuperGLUE was introduced, there was a nearly 20-point gap between the best-performing model and human performance on the leaderboard. But as of early January, two models — one from Microsoft called DeBERTa and a second from Google called T5…