IGOR: Image-GOal Representations
Atomic Control Units for Foundation Models in Embodied AI
Xiaoyu Chen†,♢, Junliang Guo†, Tianyu He†, Chuheng Zhang†, Pushi Zhang†, Derek Cathera Yang, Li Zhao†, Jiang Bian†
†Microsoft Research, ♢Tsinghua University
We introduce IGOR, a framework that learns latent actions from Internet-scale videos that enable cross-embodiment and cross-task generalization.
IGOR Framework
IGOR learns a unified latent action space for humans and robots by compressing visual changes between an image and its goal state on data from both robot and human activities. By labeling latent actions, IGOR facilitates the learning of foundation policy and world models from internet-scale human video data, covering a diverse range of embodied AI tasks. With a semantically consistent latent action space, IGOR enables human-to-robot generalization. The foundation policy model acts as a high-level controller at the latent action level, which is then integrated with a low-level policy to achieve effective robot control.
Our dataset for pretraining comprises around 2.8M trajectories and video clips, where each trajectory contains a language instruction and a sequence of observations. The datasets are curated from Open-X Embodiment (opens in new tab), Something-Something-v2 (opens in new tab), EGTEA (opens in new tab), Epic Kitchen (opens in new tab), and Ego4D (opens in new tab).
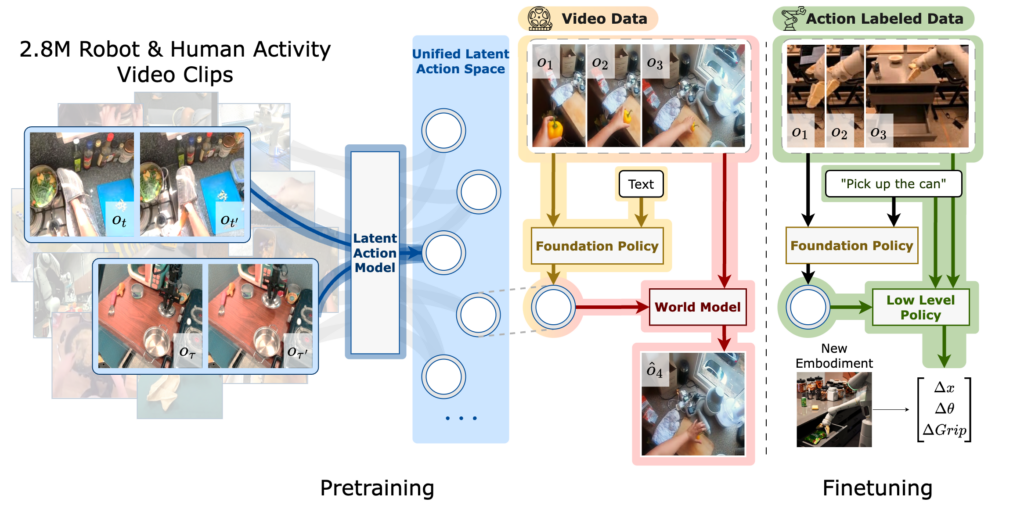
Extracting Semantically Consistent Latent Actions
IGOR learns similar latent actions for image pairs with semantically similar visual changes. On out-of-distribution RT-1 dataset, we observe that pairs with similar embeddings have similar visual changes and similar sub-tasks in semantic, for example, “open the gripper”, “move left”, and “close the gripper”. Furthermore, we observe that latent actions are shared across different tasks specified by language instructions, thereby facilitating broader generalization.
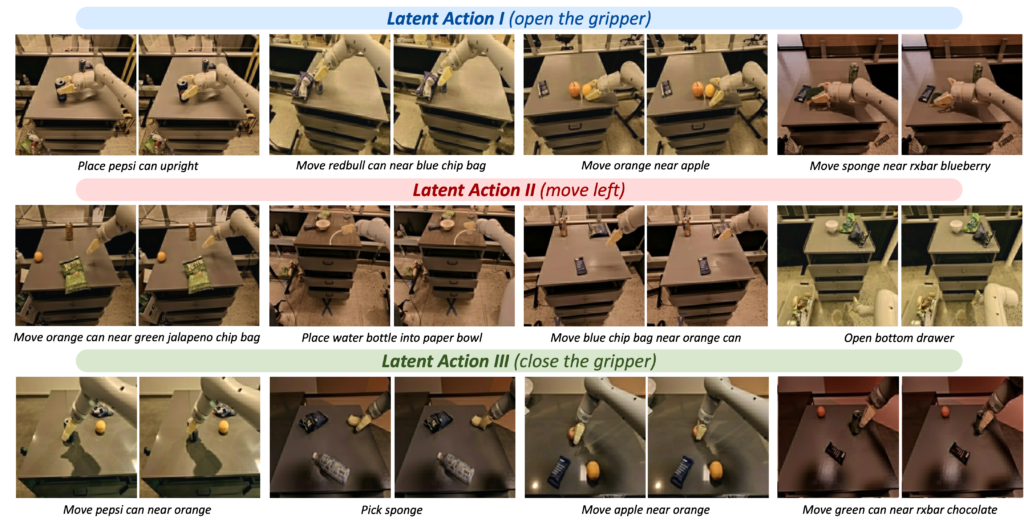
Migrating Movements Across Different Objects
IGOR successfully “migrates” the movements of objects in the one video to other videos. By applying latent actions extracted from one video, we generate new videos with similar movements on different objects with the world model. We observe that latent actions are semantically consistent across tasks with different objects.
Migrating Movements from Human to Robots
Impressively, IGOR learns latent actions that are semantically consistent across human and robots. With only one demonstration, IGOR can successfully migrate human behaviors to robot arms through only latent actions, which opens up new possibilities for few-shot human-to-robot transfer and control.
Controlling Different Objects Separately
IGOR learns to control different object’s movements separately among multiple objects. Effects of applying different latent actions are presented in the video: (a,b) move the apple, (c, d) move the tennis, and (e,f) move the orange.
Instruction Following with Policy and World Model
IGOR can follow language instructions via iteratively rolling out the foundation policy and world model. Starting from the same initial image, IGOR can generate diverse behaviors in videos that follow different instructions through latent actions.
IGOR Facilitates Learning on Low-Level Policy Model
We find that IGOR framework improves policy learning under a low-data regime on the Google robot tasks in the SIMPLER simulator shown in (a), potentially due to its capability to predict the next sub-task by leveraging internet-scale data, thereby enabling sub-task level generalization.
We also observe that image-goal pairs with similar latent actions are associated with similar low-level robot actions shown in (b). Our experiments indicate that IGOR’s learned action space reflects more information in robot movements than robot arm rotations and gripping.
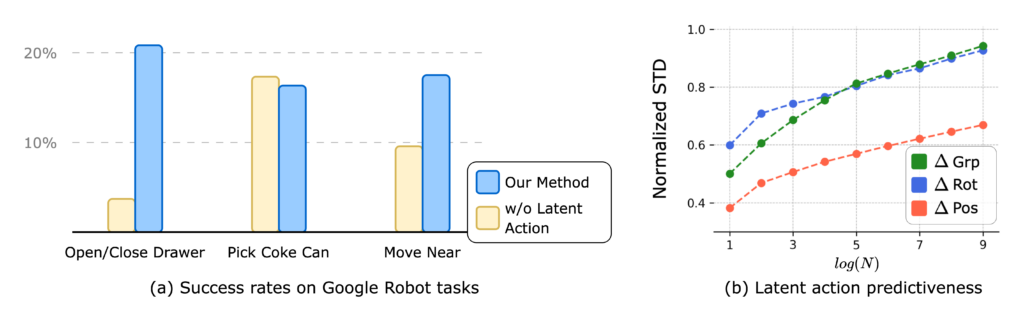
Citation
@inproceedings{chen2024igor,
title={IGOR: Image-GOal Representations are the Atomic Control Units for Foundation Model in Embodied AI},
author={Xiaoyu Chen and Junliang Guo and Tianyu He and Chuheng Zhang and Pushi Zhang and Derek Cathera Yang and Li Zhao and Jiang Bian},
year={2024},
url={https://arxiv.org/abs/2411.00785}
}