Orca is a research team in Microsoft Research. Orca focuses on creating automated pipelines for creating high-quality synthetic data at scale, and training models for specialization and model self-improvement. Orca’s research areas involve self-improvement strategies, feedback-driven teaching methods between large and small models to create high-quality synthetic data and using domain specific data to specialize LMs.
Orca focuses on the following directions:
- Automated pipelines for generating diverse and high-quality data at scale,
- Training algorithms for model specialization and continual improvement,
- Building a general pipeline for finetuning-as-a-service (automating data generation and learning for any domain).
Models
Orca: Progressive Learning from Complex Explanation Traces
Imitate reasoning processes of larger models with explanation tuning; improvements over models like Vicuna-13B by more than 100% in complex zero-shot reasoning benchmarks like Big-Bench Hard (BBH) and 42% on AGIEval.
Orca-2: Teaching Small Language Models How To Reason
Enhance smaller language models with reasoning abilities traditionally seen in larger models by teaching models to choose different strategies for varied tasks; performance levels similar or better than those of models 5-10x larger on complex tasks that test advanced reasoning abilities in zero-shot settings.
Orca models were designed for research settings, and its testing has only been carried out in such environments. It should not be used in downstream applications, as additional analysis is needed to assess potential harm or bias in the proposed application.
AgentInstruct – Creating high-quality synthetic data using agentic flows
In Orca, we recently released AgentInstruct, an extensible agentic framework for automatically creating large amounts of diverse and high-quality synthetic data. AgentInstruct can create both the prompts and responses, using only raw data sources like text documents and code files as seeds. We demonstrated the utility of AgentInstruct by creating a post training dataset of 25M pairs to teach language models different skills, such as text editing, creative writing, tool usage, coding, reading comprehension, etc. The dataset can be used for instruction tuning of any base model.
We post-trained Mistral-7b with the data. When comparing the resulting model (Orca-3) to Mistral-7b-Instruct (which uses the same base model), we observe significant improvements across many benchmarks. For example, 40% improvement on AGIEval, 19% improvement on MMLU, 54% improvement on GSM8K, 38% improvement on BBH and 45% improvement on AlpacaEval. Additionally, it consistently outperforms other models such as LLAMA-8B-instruct and GPT-3.5-turbo.
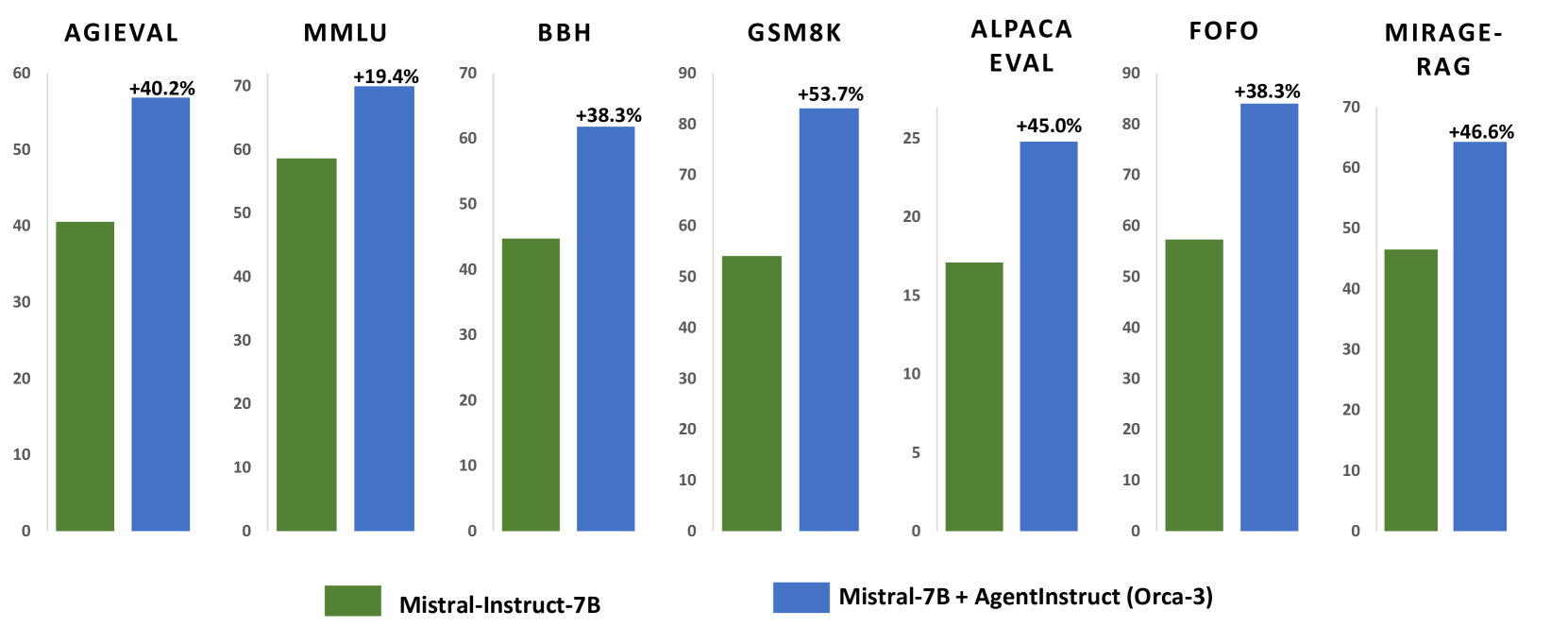