AI has transformed how we interact with technology, moving from traditional graphical interfaces to language-based, collaborative systems. To measure these new human-AI interactions, we developed Semantic Telemetry, which analyzes natural language to classify and quantify user behaviors. This method captures the context, cognition, and course of action behind user tasks, offering insights into their collaboration with AI. Our project aims to build a scalable service for data processing, with a focus on understanding both the business value and socioeconomic impact of AI.
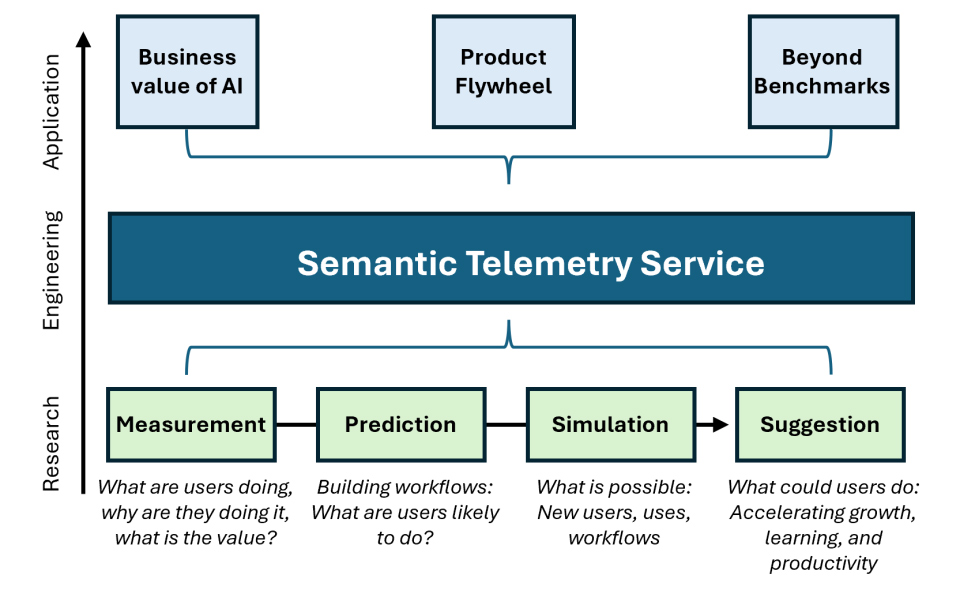
Key Takeaways:
- Big change: User-system interaction has shifted from graphical and deterministic in the PC era to language-based and probabilistic in the AI era.
- What’s needed: Understanding user behavior requires a new lexicon of what we measure and a new approach to how we measure. As a company we need clarity on the next generation of dimensions to best understand user behavior and drive a flywheel of insight and product improvement.
- Solution: Semantic Telemetry is an approach to measurement of user behavior for the AI era. With AI models at the core, the service characterizes user-AI interactions along a validated set of measures centered on user intention, cognitive process, and context.
What impact will your project have?
Currently, user-system interaction has shifted from graphical and deterministic in the PC era to language-based and probabilistic in the AI era. This project advances our ability to understand user behavior in AI systems and provides insights into key business metrics, helping to align agent-based workflows with user intentions, and providing signals for optimizing future models and experiences. This understanding will help us address long-term societal level questions about the impact of AI on knowledge work and the nature of engagement with content and information on the internet.
With this we can gain insight that would not be possible with traditional ML techniques. For example, we can leverage task intent to understand what users are trying to achieve with the conversation, whether that is image creation, text generation, information lookup, etc. to provide more context to real-world indicators like user engagement, user retention, and ad revenue.
Progress and Impact to Date – Over the past year, we have built and deployed an initial version of a Semantic Telemetry system, largely in the context of Bing Chat. This yielded a set of measurements that can classify the intents, topics, and cognitive complexity of a user’s interaction with Bing Chat and predict user retention and level of user engagement. This work has also provided insight to various business teams and has helped advance this new approach to user assessment broadly across Microsoft Copilot surfaces through our collaboration with the Learning from Interaction effort in E+D. Scientifically, our resulting analysis characterized behavioral differences in the use of traditional Bing Search and copilot-based Bing Chat, highlighting the shift in the way people are interacting with information on the internet.