News & features
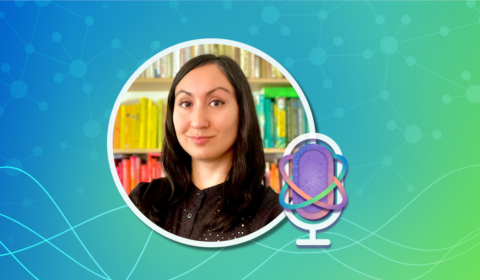
AI Frontiers: Rethinking intelligence with Ashley Llorens and Ida Momennejad
| Ashley Llorens and Ida Momennejad
Principal Researcher Ida Momennejad brings her expertise in cognitive neuroscience and computer science to this in-depth conversation about general intelligence and what the evolution of the brain across species can teach us about building AI.
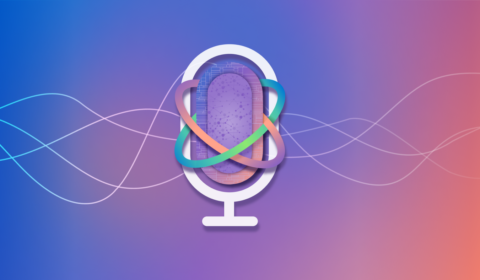
Abstracts: January 25, 2024
| Gretchen Huizinga, Jordan Ash, and Dipendra Misra
On “Abstracts,” Jordan Ash & Dipendra Misra discuss the parameter reduction method LASER. Tune in to learn how selective removal of stored data alone can boost LLM performance, then sign up for Microsoft Research Forum for more on LASER &…
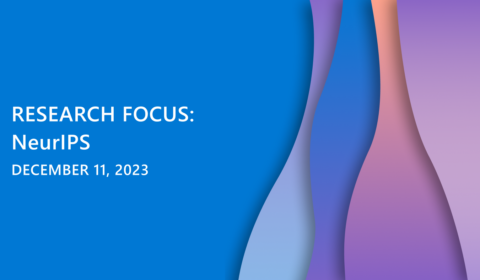
NeurIPS 2023 highlights breadth of Microsoft’s machine learning innovation
We’re proud to have 100+ accepted papers At NeurIPS 2023, plus 18 workshops. Several submissions were chosen as oral presentations and spotlight posters, reflecting groundbreaking concepts, methods, or applications. Here’s an overview of those submissions.
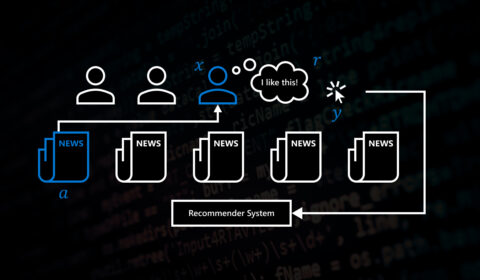
Inferring rewards through interaction
| Jessica Maghakian, Akanksha Saran, Cheng Tan, and Paul Mineiro
In reinforcement learning, handcrafting reward functions is difficult and can yield algorithms that don’t generalize well. IGL-P, an interaction-grounded learning strategy, learns personalized rewards for different people in recommender system scenarios.
Awards | International World Wide Web Conference
John Langford, Rob Schapire and co-authors receive the 2023 Seoul Test of Time Award
The International World Wide Web Conference Committee (IW3C2) announced today that the 2023 Seoul Test of Time Award will be presented to the authors of the paper “A Contextual-Bandit Approach to Personalized News Article Recommendation;” Wei Chu, (Ant Group), Lihong…
In the news | Machine Learning (Theory)
HOMER: Provable Exploration in Reinforcement Learning
Last week at ICML 2020, Mikael Henaff, Akshay Krishnamurthy, John Langford and Dipendra Misra had a paper on a new reinforcement learning (RL) algorithm that solves three key problems in RL: (i) global exploration, (ii) decoding latent dynamics, and (iii) optimizing a given…
In the news | Medium | Machine Learning
HOMER: Provable Exploration in Reinforcement Learning
At ICML 2020, Mikael Henaff, Akshay Krishnamurthy, John Langford and Dipendra Misra published a paper presenting a new reinforcement learning (RL) algorithm called HOMER that addresses three main problems in real-world RL problem: (i) exploration, (ii) decoding latent dynamics, and (iii) optimizing…
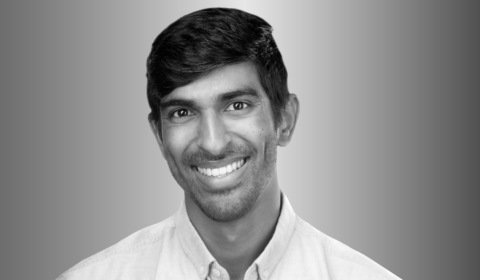
Provably efficient reinforcement learning with Dr. Akshay Krishnamurthy
MSR’s New York City lab is home to some of the best reinforcement learning research on the planet but if you ask any of the researchers, they’ll tell you they’re very interested in getting it out of the lab and…
In the news | Microsoft Research Webinar Series
Machine Learning and Fairness Webinar
In this webinar led by Microsoft researchers Jenn Wortman Vaughan and Hanna Wallach, 15-year veterans of the machine learning field, you’ll learn how to make detecting and mitigating biases a first-order priority in your development and deployment of ML systems.