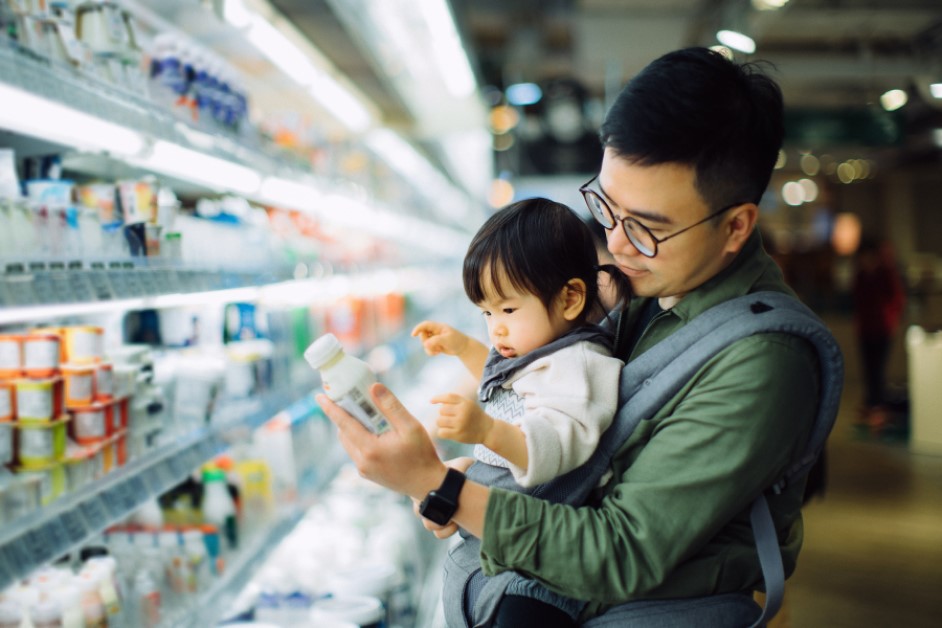
Generative AI will revolutionize Revenue Growth Management
This blog will give you an overview and a solid foundation for Revenue Growth Management (RGM). In addition, we will show you how to strategically embed generative AI to drive stronger RGM outcomes.
Revenue Growth Management is critical for revenue and profitability growth
Let’s start at the beginning. RGM is a cross-functional discipline within the Consumer-Packaged Goods (CPG) industry that uses advanced analytics to identify and analyze how to increase profits and find top-line growth opportunities. In a highly inflationary market that also experiences fast-changing consumer preferences, RGM has become a high-priority topic in most CPG organizations.
We have a bold prediction. Generative AI will give more employees access to RGM tools and insights, as well as provide a path to help companies leap from their current level to higher levels of maturity. We call this RGM democratization, and it has incredible potential.
Generative AI can address and solve some of the data, tool, and adoption challenges experienced today.
One of the largest pain points of RGM has been the speed and accuracy of insights, due to the sheer volume, complexity, and messiness of data that serve as the foundation of this discipline.
Microsoft is a trusted technology provider for many of the world’s largest CPG companies. We partner with our customers across a myriad of digital transformations. Through these experiences, we distilled the following observation of the RGM and data maturity journey for most CPGs.
Introducing the Revenue Growth Management Maturity Scale

Let’s spend some time with this chart.
At the bottom of the chart, we have the data foundations, which are essential throughout the RGM Maturity Scale. Initially, RGM projects are often one-off endeavors that involve collecting, processing, and integrating data from various sources—which can be time-consuming and challenging. The first step to take in establishing RGM maturity is to establish an RGM data hub (RGM Data Hub 1.0) and implement robust data governance practices, data cataloging, and ensure data quality and trust. The RGM data hub is then expanded by incorporating additional data sources and adopting a common data model (RGM Data Hub 2.0). The more the RGM data hub can connect with other data hubs through a connected data platform, the more value can be derived (RGM Data Hub 3.0).
RGM 1.0 represents the initial generation of RGM tools, which primarily focus on descriptive analysis of individual factors and often overlook other business drivers. These tools suffer from data latency, provide insights based on past events, have suboptimal user interfaces, and face low adoption rates.
As RGM capabilities mature, tools need to become more predictive and customized. To unlock the full potential of RGM tools, it is crucial to integrate them with planning and demand systems, and the underlying datasets must be near-real-time and more detailed (RGM 2.0).
The ultimate goal is to create an interconnected RGM system that seamlessly integrates with store-level operations at the front end, as well as the supply chain and manufacturing processes at the back end (RGM 3.0). This level of integration can only be achieved if the data foundation also evolves (RGM Data Hub 3.0) and incorporates more detailed data.
Regardless of the stage in the RGM journey, prioritizing the democratization of RGM tools is essential. Leveraging generative AI, design thinking, cross-functional decision platforms, and effective change management practices are key factors in democratizing these tools. We will be exploring the role of generative AI in democratizing RGM tools.
Generative AI is already changing how we work—making us more effective and efficient
Now, let’s discuss what generative AI is, and what it can do. Generative AI refers to a category of AI techniques and models that are designed to generate new, original content rather than simply recognizing or analyzing existing data.
Generative AI is revolutionary because it can generate the next word, an answer to a question, an effect from a cause, or a visual from a prompt, amongst many use cases. Our customers can use generative AI models with their own enterprise databases. This is unique to our Microsoft Azure OpenAI services, in addition to having the security and governance that come with a Microsoft platform.
There are four key capabilities of Azure OpenAI Services:
- Summarization of insights or scenarios
- Semantic search for knowledge mining
- Code generation
- Content generation
To learn more, please visit our Azure OpenAI Service website.
Generative AI can help companies achieve RGM maturity faster and better
Companies that use generative AI can leapfrog RGM maturity. The path no longer needs to be strictly sequential or linear. So, you might ask, what does that mean? This next section gets into more depth and details of generative AI.
RGM with a generative AI model can be enhanced through your own enterprise data and prompt engineering
A powerful way to apply generative AI for RGM is by bringing your own enterprise data and applying prompt engineering to augment the generative-pre-trained (GPT) models. Prompt engineering—also known as prompt design—involves selecting the right words, phrases, symbols, and formats that guide the model in generating high-quality and relevant texts. This involves transferring and enhancing learning with new data.
Let’s understand how this works:
- Enhancing the model with your own enterprise data: This involves connecting enterprise datasets through APIs and indexing with vector search. This process incorporates domain-specific knowledge and improves the model’s understanding of RGM concepts, terminology, and metrics—leading to more accurate and context-aware responses. This is addressed using the Azure OpenAI Service on Your Data feature.
- Enhanced context understanding: GPT models can grasp the context of RGM-related queries by experimenting with prompt engineering to optimize the models for reliability, accuracy, and safety, and generate more relevant and actionable outputs. The models can understand the relationships between pricing, promotions, sales, and other factors specific to RGM, enabling more insightful and practical recommendations.
- Personalized prompts for different personas: By providing specific prompts to GPT models, RGM professionals can guide the generated responses to align with their specific needs. Prompts can be tailored to elicit insights on pricing strategies, promotional effectiveness, assortment planning, or any other relevant aspect of RGM.
- Bias mitigation: The responsible AI framework provides an opportunity to address biases that may exist in the base GPT model. RGM professionals can curate diverse training datasets and incorporate ethical considerations to ensure fair and unbiased recommendations on areas like pricing strategies or assortment resets. The Microsoft journey towards responsible AI began nearly six years ago now, with Satya Nadella penning an article in Slate magazine titled “The partnership of the future.” Learn more about Microsoft’s responsible AI principles.
- Improved insights and decision-making: GPT models using RGM-specific data can enhance performance on RGM tasks. The models can learn from historical RGM data, market trends, and industry best practices—allowing for more nuanced and contextual insights leading to better decision-making on domains like demand forecasting, pricing optimization, and promotional strategy.
It’s important to note that this experimentation and operationalization process requires a well-curated dataset, expertise in RGM, and careful consideration of privacy and ethical concerns. Additionally, ongoing monitoring and evaluation are necessary to ensure the models continue to provide reliable and relevant guidance in the dynamic RGM space.
Increasing speed and access to RGM insights through generative AI
The use of generative AI will change how people use existing tools and improve productivity across the RGM ecosystem—from planning, execution, and optimization. Today the use of RGM digital tools is limited to a few data analysts and power users. For business users, the UI, UX, and algorithm logic are not easy to understand or use. Generative AI makes the UX simpler, giving business users the ability to use natural language prompts with nuanced and complex questions to extract relevant and contextual insights and scenarios, summarize them, and quickly share those insights with cross-functional partners and customers.
For example:
- A trade promotional manager at a beverage company would have the ability to be very surgical in allocating promotional budgets to specific retailers, promotional types, brands, and SKUs by asking questions like: “Give me options on how and what to promote and at what frequency to get a promotional ROI of greater than 1.5 in the greater Chicago area in club stores in the summer months of May to September.”
- A channel pricing manager can assess revenue loss risk-based pricing with natural language questions like: “In my sales district, is my (Brand A) priced competitively versus (Brand B) across all stores? Show me the stores where I have the maximum risk of losing sales based on price gaps.”
- A store manager would have the ability to plan store shelves better in case of out-of-stock scenarios by asking: “What is the best SKU to substitute if I am out of stock on the small size of (Brand X) so that I can mitigate category sales loss, considering inventory levels available in the store back room?”
Data Science teams will be more productive with these models
Data Science teams use statistical modeling and machine learning models to understand events and to predict trends by observing the cause and effect using data that is available to them. These models are not designed to be repeatable and scalable in most cases and often suffer from poor documentation. With generative AI, Data Science teams build code for the predictive machine learning models that result in increased productivity. Generative AI can better organize data, pick up anomalies, assist in code generation, and document code for faster, better, and more reusable code generation. This can increase productivity and scalability across the data science discipline.
Bringing it all together—generative AI will lead to RGM democratization
The RGM Maturity Scale is a way for CPG companies to gauge where they are on the data integration journey. The ultimate goal is to have a system that is fully integrated where data flows from both front and back-end processes. Generative AI can help companies leap from one level to another.
Generative AI can be applied to RGM through your own enterprise data and prompt engineering. This can lead to more accurate and context-aware responses. It can provide insights to guide overall strategies, limit bias, and improve the performance of RGM tasks. Using generative AI will change how people use existing tools. Productivity can be increased when usable insights are provided to CPG leaders faster.
Incorporating generative AI can propel CPG companies toward their RGM goals. With generative AI, it is possible for companies to leap entire steps in the RGM Maturity Scale. This will result in finding top-line growth opportunities and increased profits.
How to get started
- Assess your company’s current RGM and data capabilities using the Microsoft RGM and Data Maturity Scale.
- Set your RGM and data foundation goals aligned to business priorities.
- Create a comprehensive roadmap with data, technology, AI, and change management dimensions.
- Assess how you would like to apply generative AI to your RGM goals no matter where you are in terms of RGM maturity.
- Take small steps towards adopting generative AI, starting with a use case or an objective.
- Develop and deploy responsible AI measures from the start.
Microsoft and our partner ecosystem can meet your organization at any maturity stage, including getting started or scaling your existing initiatives to priority business segments or markets.
Learn more
- Azure OpenAI Service homepage
- Microsoft for Consumer Goods homepage
- Microsoft is helping consumer goods brand marketers embrace the era of AI
- The era of AI: How the Microsoft Cloud is accelerating AI transformation across industries
- Reflections on AI and the future of human flourishing
- Announcing Microsoft’s AI Customer Commitments
- Microsoft’s framework for building AI systems responsibly
- Microsoft and responsible AI
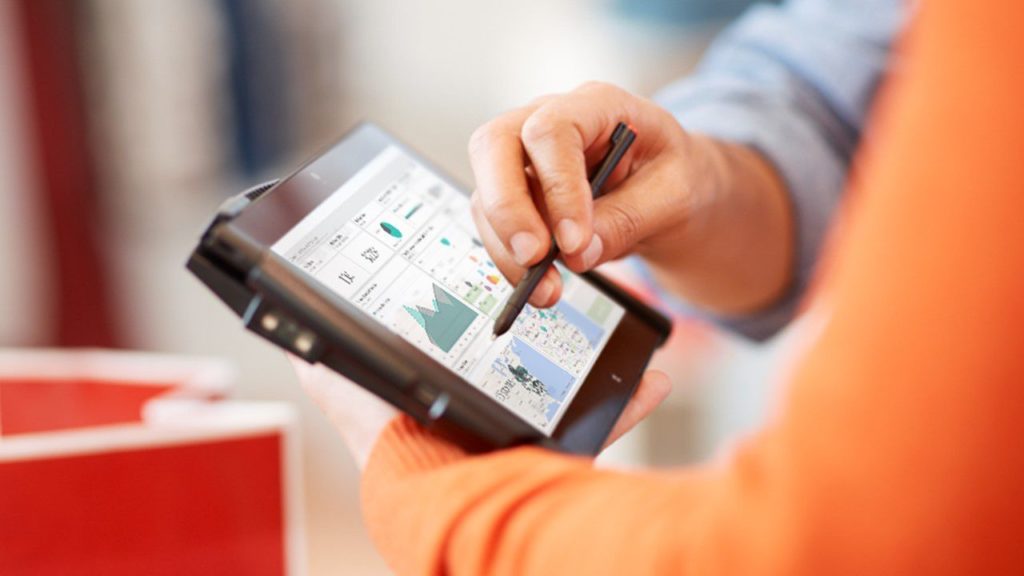