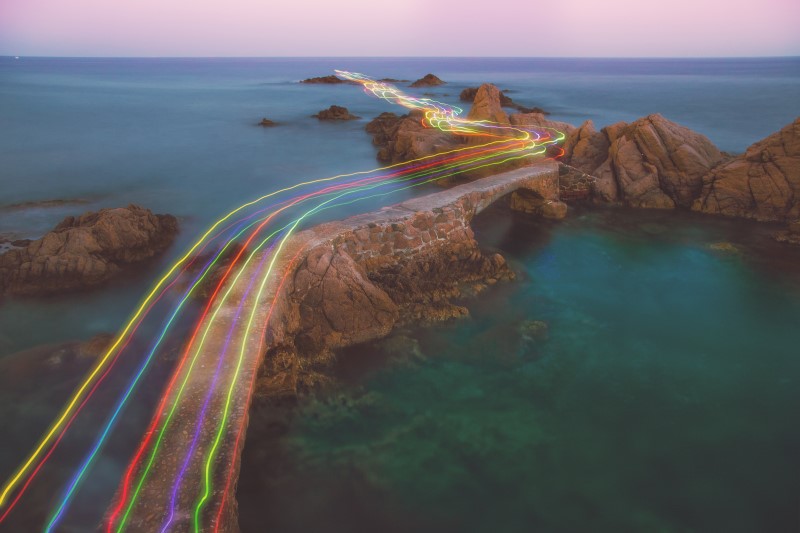
From discussion to deployment: 4 key lessons in generative AI
A year ago, few had heard the term ‘generative AI’, but with the launch of OpenAI’s ChatGPT that all changed very rapidly. Within 64 days of launch, ChatGPT had over 100 million users1 and this interest changed completely the conversations between business teams and their IT counterparts.
It also changed the conversations Microsoft customer-facing teams were having as organizations scrambled to exploit the technology. There are very few customer conversations and major projects where some form of generative AI does not have a part to play.
Microsoft teams have worked hard to advise customers on the best way forward and there have been lessons learned from all sides. Conversations are now starting to change, however. They are moving away from being primarily focused on ‘What can we do with generative AI?’ toward ‘How should we approach generative AI?’.
There are some lessons that have been learned in customer interactions that are now being applied to projects to maximize the return on investment for our customers. Each of these four areas you may wish to give consideration to as you explore possibilities within your organization.
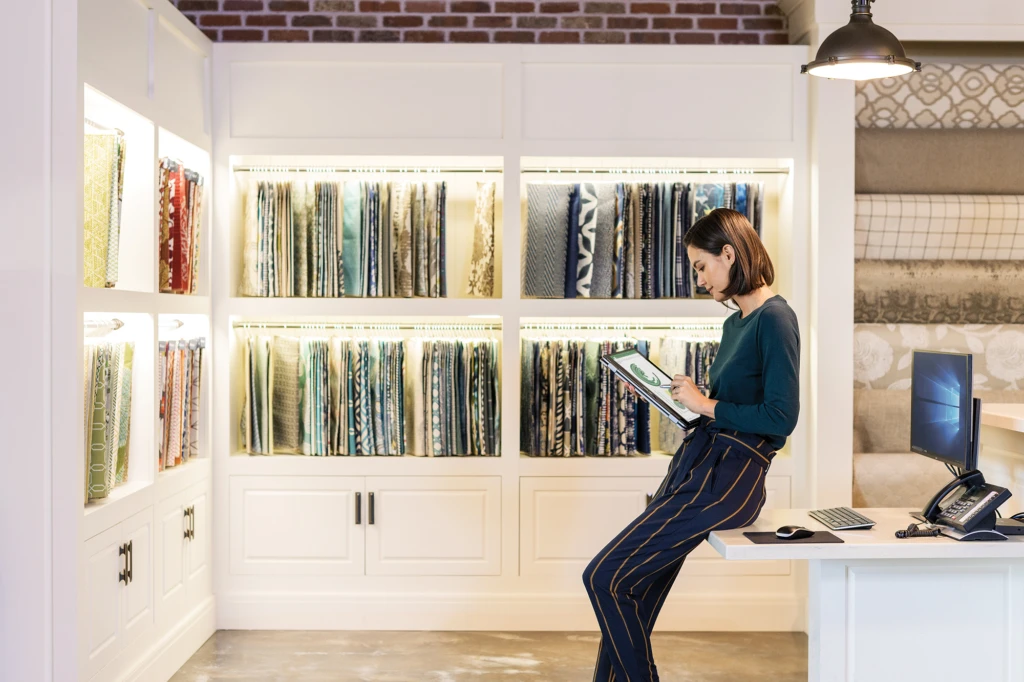
1. Be clear on the problem
There can be a temptation to see generative AI as if it were a ‘new toy’ waiting to be played with. It is critical however to be focused on the problem you are trying to solve and to use this to ‘paint the vision’ of what you want to achieve.
An approach that can work well is to identify several candidate initiatives or use cases where generative AI may be able to help. Examining these more closely could reveal that there are other ways to solve some of them. There may also be some that you can cluster together because they could warrant similar approaches to address their needs.
Prioritizing based on likely business impact versus the effort required is an excellent way to determine which use case, or use cases, you should start with. This is a technique Microsoft Industry Architects use with customers engaging with both technical and business teams at the same time. Placing each use case on a simple four-quadrant matrix with ‘Effort’ on the x-axis and ‘Impact’ on the y-axis gives a simple yet effective visualization approach to setting priorities.
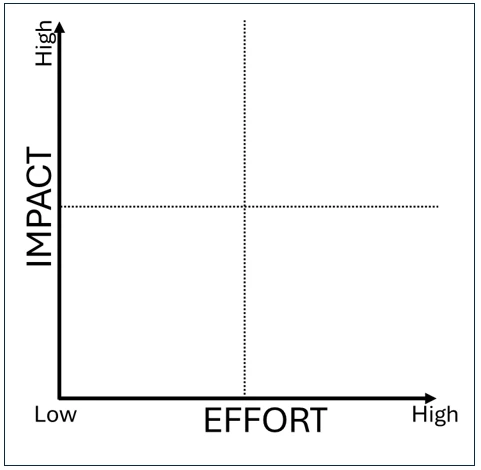
When considering impact, consider things like speed or time saving, improved decision making, and cost savings but also consider how long it will take to reap the benefits. When considering effort, think about the time and money you would need to invest to achieve the desired outcome while being conscious of the different systems and personas that would be involved.
Once you have determined your target use case or use cases, you must be clear about what ‘good’ looks like. This means determining the metrics and values by which you will measure your success. Using quantitative terms can deliver real focus. Your metric could be based on time, effort, or money—but try to make it as measurable and meaningful as possible.
2. Work inside-out
As a customer-centric organization, it might feel counter-intuitive to focus internally first. With new innovative technologies like generative AI, this is a great way to try out the technology and your approaches on a ‘friendly’ audience—your own internal teams—before applying to your external customers.
This also acts as the foundation for a roadmap of projects and initiatives all centered around generative AI. You can think of this roadmap in three phases—which you could align to a ‘Crawl-Walk-Run’ approach.
Phase 1: Crawl
Use cases inside the organization
This focuses on ‘human in the loop’ reviews of content that is generated. In a retail and consumer goods environment example use cases might include:
- Summarization and analysis—call center and customer interaction summarization.
- Categorization—knowledge management and internal communications.
- Content generation—creating product images for your e-commerce platform or creating product descriptions based on multiple inputs.
Phase 2: Walk
Use cases that directly interact with employees and customers
In this phase, you may have human supervision of multiple use cases, each providing information to help the employee or customer, human, make better-informed decisions. Retail and consumer goods examples might include:
- Summarization and analysis—summarizing product reviews, product descriptions, and specifications. A great example of this is how CarMax is creating their used car listings—saving 11 years of content generation effort.
- Categorization—analyzing social media trends, sentiment analysis, or making product design recommendations.
- Content generation—personalized user experience or marketing campaign content generation.
Phase 3: Run
Increased automation
This final phase focuses on new product offerings or automation that you might provide as direct interaction with your customers. Examples in a retail or consumer goods setting could include:
- Summarization and analysis—call-center automation and the contextual automation of customer-facing business processes.
- Categorization—automating the creation of product descriptions for your commerce platform considering consumer feedback.
- Content generation—automated creation of personalized marketing and brand content.
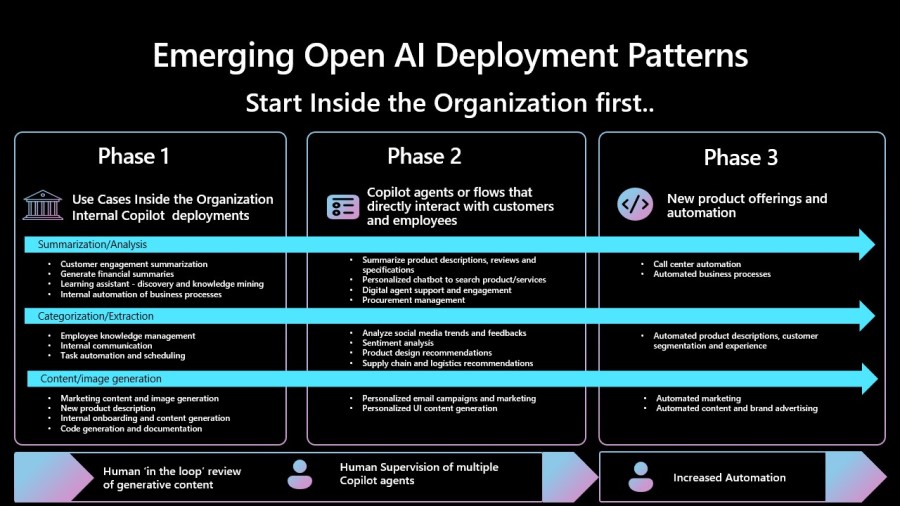
3. Be business (problem) led
Prioritizing use cases based on effort versus impact is likely to ensure you are maximizing your focus on a problem that can deliver real value for your business. ‘Painting the vision’ of what you are trying to achieve remains key here.
Ongoing input from business teams remains key—like all initiatives where technology plays a major part, the most successful initiatives tend to be where technology and their business counterparts work most closely—all focused on the specific business problem.
In any technology-focused initiative, people, processes, and technology all need to be considered together and generative AI is no different. Consequently, it is important to not ignore specific elements. For example, as part of your work focused on each use case you should ensure you:
Microsoft responsible ai
Explore our perspective- Determine the business process associated with your use case. Understand the triggers that will initiate it along with the points of interaction with different systems and personas.
- Establish clarity on the personas that interact with the process but also understand who will be impacted when your use case is successfully delivered and used in the business environment.
- Establish how personas who need to be involved will interact with the process—establish when and how they will need to be notified of interaction or approval they need to undertake. This is especially important when considering the responsible AI principle of accountability.
- Look carefully at your use case from a responsible AI perspective. Using tools like the Microsoft Responsible Impact Assessment could accelerate things for you here.
- Change management should be examined carefully. Understanding the personas impacted is essential to aid this. Given how new generative AI is and the large amount of information in the media there are sensitivities that may require careful handling. Again, careful application and consideration of the Microsoft responsible AI principles of fairness, reliability and safety, privacy and security, inclusiveness, transparency, and accountability will help you in this regard.
4. Equip yourself (to follow fast)
It is good to be excited about the potential of generative AI—according to McKinsey the productivity it could add to the global economy equates to $2.6 trillion to $4.4 trillion annually.2 These numbers are significant given that in 2021 the GDP of the United Kingdom was $3.1 trillion. Consequently, it is important to be able to innovate using this technology.
To do this, you need to build skills inside your own organization to be able to deliver use cases quickly. This means nurturing your own teams and in-house capabilities.
It could be very tempting to adopt a ‘fast follower’ approach where if you see a competitor launch a generative AI-powered solution that aids their customers you rapidly follow with something similar—or perhaps even better.
There is nothing wrong with this as a strategy, but it needs the in-house skills and experience to have to be nurtured over time to have the capability to deliver against this. Delivering use cases you have prioritized and innovated against is one of the best ways to nurture the in-house skills you need.
By reaching 100 million users in 64 days, ChatGPT became the fastest-growing consumer application in history.3 This potentially sets the scene for generative AI to become the fastest-growing technology category ever seen.
While the overall space in terms of possibilities and benefits is likely to evolve rapidly, looking carefully at these four areas will help your organization learn while effectively harnessing the power of generative AI to deliver value for your business.
Learn more
- Microsoft Cloud for Retail
- Generative AI will revolutionize Revenue Growth Management
- “What’s my generative AI strategy?” Microsoft is helping consumer goods brand marketers embrace the era of AI
- How next-generation AI and digital tools transform the retail frontline
- Microsoft’s responsible AI principles
- CarMax puts customers first with car research tools powered by Azure OpenAI Service
- Welcoming the generative AI era with Microsoft Azure
- Generative AI transforms business processes
1 The Guardian, ChatGPT reaches 100 million users two months after launch, February 2023.
2 McKinsey Digital, The economic potential of generative AI: The next productivity frontier, June 2023.
3 Reuters, ChatGPT sets record for fastest-growing user base—analyst note, February 2023.